Brain signals from a primate directly move paralyzed limbs in another primate ‘avatar’
February 24, 2014
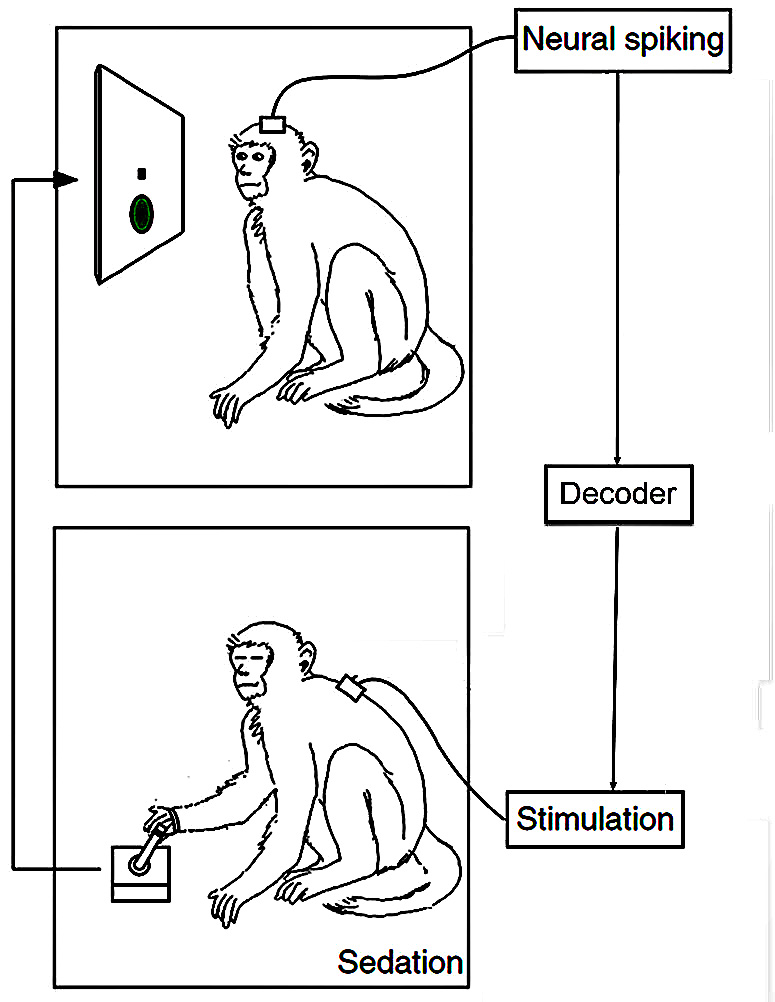
Neural activity signals recorded from pre-motor neurons (top) are decoded and played back to control limb movements in a functionally paralyzed primate avatar (bottom) — a step toward making brain-machine interfaces for paralyzed humans to control their own limbs using their brain activity alone (illustration adapted) (credit: Maryam M. Shanechi et al./Nature Communications)
Taking brain-machine interfaces (BMI) to the next level, new research may help paralyzed people move their own limb just by thinking about it.
Previous research has been limited to controlling external devices, such as robots or synthetic avatar arms.
In a paper published online Feb. 18 in Nature Communications, Maryam Shanechi, assistant professor of electrical and computer engineering at Cornell University, working with Ziv Williams, assistant professor of neurosurgery at Harvard Medical School, and colleagues describe a cortical-spinal prosthesis that directs “targeted movement” in paralyzed limbs.
The research team developed and tested a prosthesis that connects two subjects (monkeys) by enabling one subject to send its recorded neural activity to control limb movements in a different subject that is temporarily sedated. The demonstration is a step forward in making brain-machine interfaces for paralyzed humans to control their own limbs using their brain activity alone.
The concept: when paralyzed patients imagine or plan a movement, neurons in the brain’s motor cortical areas still activate, even though the communication link between the brain and muscles is broken. By implanting sensors in these brain areas, neural activity could be recorded and translated to the patient’s desired movement using a mathematical transform called the decoder. These interfaces could allow patients to generate movements directly with their thoughts.
Decoding algorithms
The brain-machine interface in the experiment is based on a set of real-time decoding algorithms that process neural signals by predicting their targeted movements. In the experiment, one animal acted as the controller of the movement (the “master”). That animal “decided” which target location to move to, and generated the neural activity. The decoded movement was then used to directly control the limb of the other animal by electrically stimulating its spinal cord.
“The problem here is not only that of decoding the recorded neural activity into the intended movement, but also that of properly stimulating the spinal cord to move the paralyzed limb according to the decoded movement,” Shanechi said.
The scientists focused on decoding the target endpoint of the movement as opposed to its detailed kinematics (the exact sequence of movements). This allowed them to match the decoded target with a set of spinal stimulation parameters that generated limb movement toward that target. They demonstrated that the alert animal (the master) could produce two-dimensional movement in the sedated animal’s limb — a breakthrough in brain-machine interface research.
“By focusing on the target end point of movement as opposed to its detailed kinematics, we could reduce the complexity of solving for the appropriate spinal stimulation parameters, which helped us achieve this 2-D movement,” Williams said.
Part of the experimental setup’s novelty was using two different animals, rather than just one with a temporarily paralyzed limb. That way, the scientists contend that they have a true model of paralysis, since the master animal’s brain and the sedated animal’s limb had no physiological connection, as is the case for a paralyzed patient.
“The next step is to advance the development of brain-machine interface algorithms using the principles of control theory and statistical signal processing,” Shanechi said. “Such brain-machine interface architectures could enable patients to generate complex movements using robotic arms or paralyzed limbs.”
Abstract of Nature Communications paper
Motor paralysis is among the most disabling aspects of injury to the central nervous system. Here we develop and test a target-based cortical–spinal neural prosthesis that employs neural activity recorded from premotor neurons to control limb movements in functionally paralysed primate avatars. Given the complexity by which muscle contractions are naturally controlled, we approach the problem of eliciting goal-directed limb movement in paralysed animals by focusing on the intended targets of movement rather than their intermediate trajectories. We then match this information in real-time with spinal cord and muscle stimulation parameters that produce free planar limb movements to those intended target locations. We demonstrate that both the decoded activities of premotor populations and their adaptive responses can be used, after brief training, to effectively direct an avatar’s limb to distinct targets variably displayed on a screen. These findings advance the future possibility of reconstituting targeted limb movement in paralysed subjects.