‘Brain-to-Text’ system converts speech brainwave patterns to text
June 16, 2015
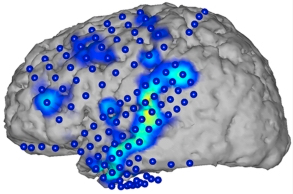
Brain activity recorded by electrocorticography electrodes (blue circles). Spoken words are then decoded from neural activity patterns in the blue/yellow areas. (credit: CSL/KIT)
German and U.S. researchers have decoded natural continuously spoken speech from brain waves and transformed it into text — a step toward communication with computers or humans by thought alone.
Their “Brain-to-Text” system recorded signals from an electrocorticographic (ECoG)* electrode array located on relevant surfaces of the frontal and temporal lobes of the cerebral cortex of seven epileptic patients, who participated voluntarily in the study during their clinical treatment.
The patients read sample text (from a limited set of words) aloud during the study. Machine learning algorithms were then used to extract the most likely word sequence from the signals, and automatic speech-to-text methods created the text output. The system achieved word error rates as low as 25% and phone (instances of phonemes in utterances) error rates below 50%.
The researchers suggest that the Brain-to-Text system might lead to a speech-communication method for locked-in (unable to communicate) patients in the future.
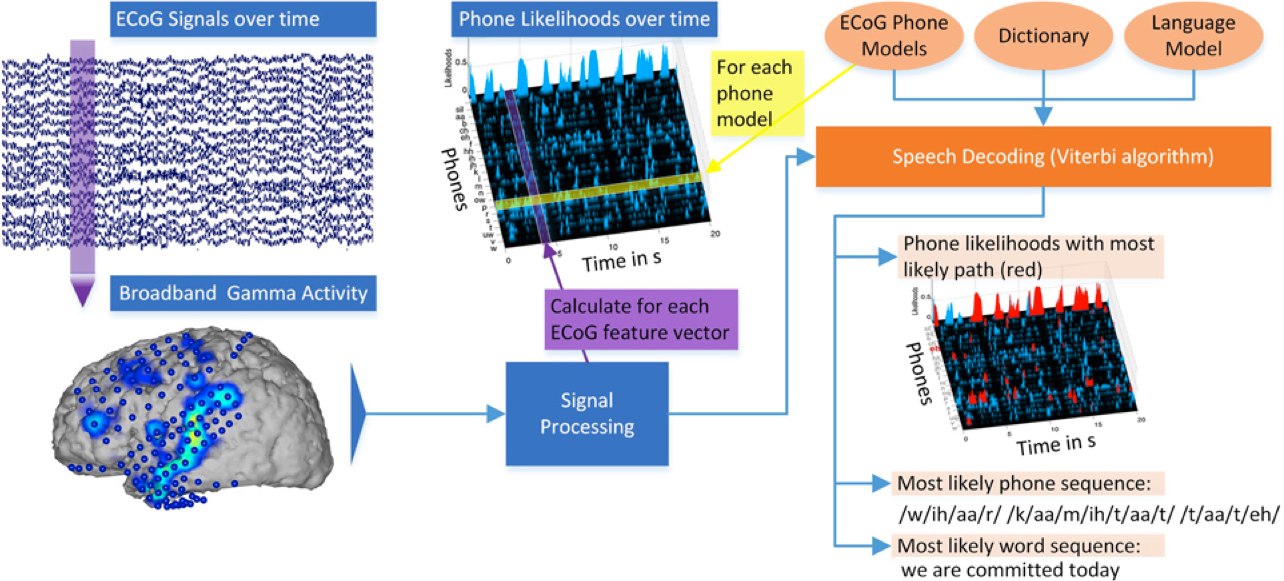
Overview of the Brain-to-Text system: ECoG broadband gamma-wave signals for every electrode are recorded. Stacked broadband gamma features are calculated by signal processing. Phone (phoneme sounds) likelihoods over time are calculated by evaluating all Gaussian ECoG phone models for every segment of ECoG features. Using ECoG phone models, a dictionary and an n-gram language model, phrases are decoded using the Viterbi algorithm. The most likely word sequence and corresponding phone sequence are calculated and the phone likelihoods over time can be displayed. Red marked areas in the phone likelihoods show most likely phone path. (credit: CSL/KIT)
The open-access research was conducted by an interdisciplinary collaboration of informatics, neuroscience, and medicine researchers. The brain recordings were conducted at Albany Medical Center (Albany, NY). The signal processing and automatic speech recognition methods were developed at the Cognitive Systems Lab of Karlsruhe Institute of Technology (KIT) in Germany.
This work was supported by the NIH, the US Army Research Office, and Fondazione Neuron, and received support by the International Excellence Fund of Karlsruhe Institute of Technology, Deutsche Forschungsgemeinschaft, and Open Access Publishing Fund of Karlsruhe Institute of Technology.
* ECoG technology uses a dense array of needles to record signals directly from neurons at high spatial resolution, high temporal (time) resolution, and high signal-to-noise ratio.
Abstract of Brain-to-text: decoding spoken phrases from phone representations in the brain
It has long been speculated whether communication between humans and machines based on natural speech related cortical activity is possible. Over the past decade, studies have suggested that it is feasible to recognize isolated aspects of speech from neural signals, such as auditory features, phones or one of a few isolated words. However, until now it remained an unsolved challenge to decode continuously spoken speech from the neural substrate associated with speech and language processing. Here, we show for the first time that continuously spoken speech can be decoded into the expressed words from intracranial electrocorticographic (ECoG) recordings.Specifically, we implemented a system, which we call Brain-To-Text that models single phones, employs techniques from automatic speech recognition (ASR), and thereby transforms brain activity while speaking into the corresponding textual representation. Our results demonstrate that our system can achieve word error rates as low as 25% and phone error rates below 50%. Additionally, our approach contributes to the current understanding of the neural basis of continuous speech production by identifying those cortical regions that hold substantial information about individual phones. In conclusion, the Brain-To-Text system described in this paper represents an important step toward human-machine communication based on imagined speech.