Brains that switch between active areas more often learn faster
April 20, 2011
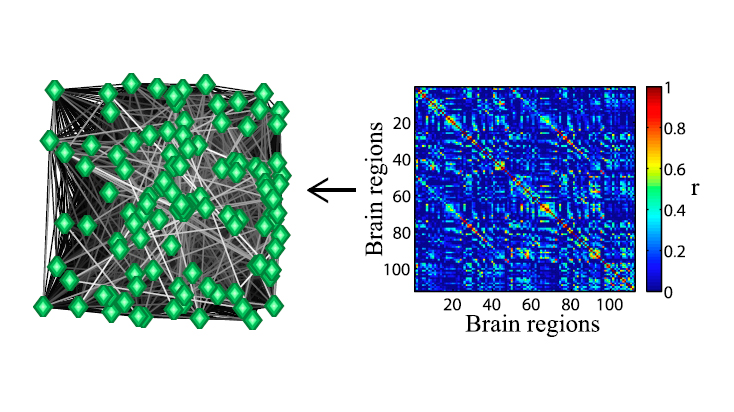
The functional connectivity between two cortical structures is given by a Pearson correlation between the mean regional activity of fMRI signals from 112 brain regions (right). The resulting N × N correlation matrix was statistically corrected by constructing a subject-specific weighted functional brain network (left). (Credit: Danielle S. Bassett et al./PNAS)
The “flexibility” of a person’s brain — how much different areas of the brain link up in different combinations — can be used to predict how fast someone will learn, according to research by an international team from Oxford University, UC Santa Barbara, and UNC Chapel Hill.
The team ran an experiment over 3 sessions in which 18 volunteers had to push a series of buttons as fast as possible. They then divided functional MRI images of each volunteer’s brain into 112 different regions and analyzed how these different areas were active together while they performed the task.
They found that people with more “flexible” brains, whose brain regions switched active areas more often, were faster at learning the motor tasks.
“It’s the first time that anyone has defined this concept of ‘flexibility’ in the brain: how brain regions ‘light up’ together in different combinations. We’ve been able to show that how much these areas ‘swap partners’ in one session can predict how fast people will perform a task in a later session,” said Dr Mason Porter of Oxford University’s Mathematical Institute, an author of the report. “It suggests that in order to learn, the networks of our brains have to be flexible.”
The new study uses computational methods developed to analyze what the researchers call “multilayer” networks, in which each “layer” might represent a network at one snapshot in time or a different set of connections between the same set of regions or individuals.
These layers are combined into a larger mathematical object, which can contain a potentially huge amount of data and is difficult to analyse. Previous methods could only deal with each layer separately, and it was necessary to compare the results obtained from different layers using ad hoc tools (if it was possible at all) and the new method overcomes this key challenge.
“With this technique, we are treating the brain like a social network in which each of the 112 regions is analogous to a person, then looking at how they interact with each other over time,” said Porter. One of the reasons the study is small is that, even taking fMRI data from a small number of individuals over three sessions, this turns out to be an immense computing task: this project included 10,000 days of computing (CPU) time to process, though we’re now working on making our computations more efficient to be able to handle larger amounts of data from experiments.”
Ref.: Danielle S. Bassett et al., Dynamic reconfiguration of human brain networks during learning, PNAS, April 18, 2011, doi:10.1073/pnas.1018985108