Cameras talk to each other to identify, track people
November 13, 2014

Frames from a moving camera recorded by the Swiss Federal Institute of Technology show how UW technology distinguishes among people by assigning each person a unique color and number, then tracking them as they walk (credit: Swiss Federal Institute of Technology)
University of Washington electrical engineers have developed a way to automatically track people across moving and still cameras by using an algorithm that trains the networked cameras to learn one another’s differences. The cameras first identify a person in a video frame, then follow that same person across multiple camera views.

The tracking system first systematically picks out people in a camera frame, then follows each person based on his or her clothing texture, color and body movement (credit: Swiss Federal Institute of Technology)
“Tracking humans automatically across cameras in a three-dimensional space is new,” said lead researcher Jenq-Neng Hwang, a UW professor of electrical engineering. “As the cameras talk to each other, we are able to describe the real world in a more dynamic sense.”
Hwang and his research team presented their results last month in Qingdao, China, at the Intelligent Transportation Systems Conference sponsored by the Institute of Electrical and Electronics Engineers (IEEE).
With the new technology, a car with a mounted GPS display could take video of a scene, then identify and track humans and overlay them on a virtual 3D map on the display. The UW researchers are developing this to work in real time, which could track a specific person who is dodging the police.
Real-time tracking by Google Earth
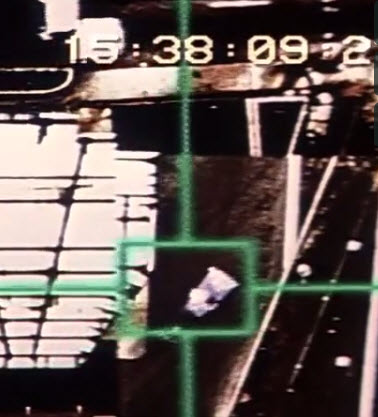
NSA satellite tracking, from “Enemy of the State” movie (credit: Buena Vista Pictures)
“Our idea is to enable the dynamic visualization of the realistic situation of humans walking on the road and sidewalks, so eventually people can see the animated version of the real-time dynamics of city streets on a platform like Google Earth,” Hwang said.
Hwang’s research team in the past decade has developed a way for video cameras — from the most basic models to high-end devices – to talk to each other as they record different places in a common location.
The problem with tracking a human across cameras of non-overlapping fields of view is that a person’s appearance can vary dramatically in each video because of different perspectives, angles and color hues produced by different cameras.
The researchers overcame this by building a link between the cameras. Cameras first record for a couple of minutes to gather training data, systematically calculating the differences in color, texture and angle between a pair of cameras for a number of people who walk into the frames in a fully unsupervised manner without human intervention.
After this calibration period, an algorithm automatically applies those differences between cameras and can pick out the same people across multiple frames, effectively tracking them without needing to see their faces.
The research team has tested the ability of static and moving cameras to detect and track pedestrians on the UW campus in multiple scenarios. In one experiment, graduate students mounted cameras in their cars to gather data, then applied the algorithms to successfully pick out humans and follow them in a three-dimensional space.
Robot and drone-based tracking
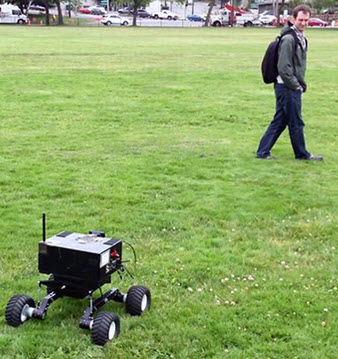
A robot equipped with a camera follows a researcher by tracking him as he walks (credit: U of Washington)
They also installed the tracking system on cameras placed inside a robot and a flying drone, allowing the robot and drone to follow a person, even when the instruments came across obstacles that blocked the person from view.
The linking technology can be used anywhere, as long as the cameras can talk over a wireless network and upload data to the cloud.
The researchers say this detailed visual record could be useful for security and surveillance, monitoring for unusual behavior or tracking a moving suspect. But it also tells store owners and business proprietors useful information and statistics about consumers’ moving patterns.
A store owner could, for example, use a tracking system to watch a shopper’s movements in the store, taking note of her interests. Then, a coupon or deal for a particular product could be displayed on a nearby screen or pushed to the shopper’s phone.
Or a government could track terrorists (or other persons of interest).
The research was funded by the Electronics and Telecommunications Research Institute of Korea and the UW Applied Physics Laboratory.
University of Washington | Cameras talk to each other to track pedestrians
Enemy of the State Showreel from Filament Post on Vimeo
Abstract of Driving Recorder Based On-Road Pedestrian Tracking Using Visual SLAM and Constrained Multiple-Kernel
The proposed system systematically detects the pedestrians from recorded video frames and tracks the pedestrians in the V-SLAM inferred 3-D space via a tracking-by-detection scheme. In order to efficiently associate the detected pedestrian frame-by-frame, we propose a novel tracking framework, combining the Constrained Multiple-Kernel (CMK) tracking and the estimated 3-D (depth) information, to globally optimize the data association between consecutive frames. By taking advantage of the appearance model and 3-D information, the proposed system not only achieves high effectiveness but also well handles occlusion in the tracking. Experimental results show the favorable performance of the proposed system which efficiently tracks on-road pedestrian in a moving camera equipped on a driving vehicle.
Abstract of Fully Unsupervised Learning of Camera Link Models for Tracking Humans Across Nonoverlapping Cameras
A multiple-camera tracking system that tracks humans across cameras with nonoverlapping views is proposed in this paper. The systematically estimated camera link model, including transition time distribution, brightness transfer function, region mapping matrix, region matching weights, and feature fusion weights, is utilized to facilitate consistently labeling the tracked humans. The system is divided into two stages: in the training stage, based on an unsupervised scheme, we formulate the estimation of the camera link model as an optimization problem, in which temporal features, holistic color features, region color features, and region texture features are jointly considered. The deterministic annealing is applied to effectively search the optimal model solutions. The unsupervised learning scheme tolerates the presence of outliers in the training data well. In the testing stage, the systematic integration of multiple cues from the above features enables us to perform an effective reidentification. The camera link model can be continuously updated during tracking in the testing stage to adapt the changes of the environment. Several simulations and comparative studies demonstrate the superiority of our proposed estimation method to the others. Moreover, the complete system has been tested in a small-scale real-world camera network scenario.