Computational biomarkers can identify at-risk heart attack victims
September 29, 2011
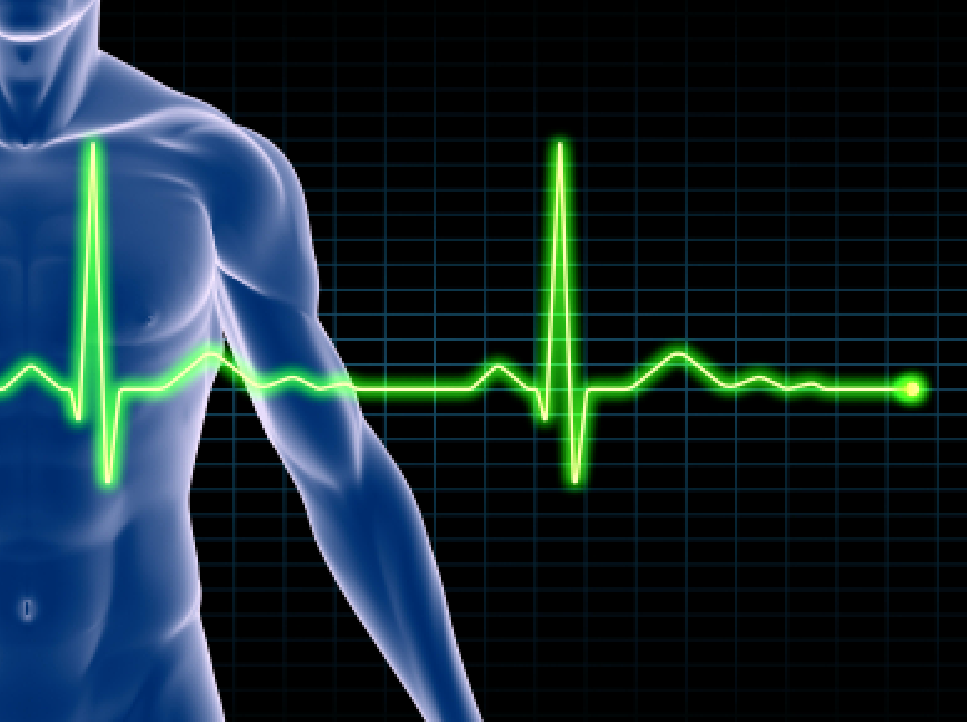
EKG electrocardiogram (credit: stock image)
Subtle markers of heart damage hidden in plain sight among hours of EKG recordings could help doctors identify which heart attack patients are at high risk of dying soon, researchers from the University of Michigan, MIT, Harvard Medical School, and Brigham and Women’s Hospital in Boston have discovered,
The findings could help match tens of thousands of cardiac patients with life-saving treatment in time. Approximately 1 million Americans have a heart attack each year. In certain age groups, more than a quarter of those who survive the initial attack end up dying of complications within a year, according to the American Heart Association.
Missing 70 percent of high-risk patients
“Today’s methods for determining which heart attack victims need the most aggressive treatments can identify some groups of patients at a high risk of complications. But they miss most of the deaths — up to 70 percent of them,” said Zeeshan Syed, an assistant professor in the U-M Department of Electrical Engineering and Computer Science and first author of the study.
Using data mining and machine learning techniques, the researchers sifted through 24-hour continuous electrocardiograms (EKGs or ECGs) from 4,557 heart attack patients. They found that the EKG signals from many of the patients who later suffered cardiovascular death contained similar errant patterns that until now were dismissed as noise or simply undetectable. EKGs are monitored continually while a patient is hospitalized, but it’s difficult for doctors to determine the most important predictors of outcome from these long recordings. They typically look at its data in snapshots that are only a few seconds long.
Today, doctors prescribe the most aggressive post-heart attack treatments based on several factors including the patient’s overall health, medical history, the results of several blood tests, and an echocardiogram. The echocardiogram (different from an electrocardiogram) uses ultrasound to image the heart and measure how much blood it pumps through with each beat.
“Unfortunately, it is a rather blunt metric and we tend to miss a fair number of the patients with the sickest hearts,” said Benjamin Scirica, a cardiologist at Brigham and Women’s Hospital who was also involved in the study. “We have to do a better job of identifying patients at high risk.”
During the months after a heart attack, patients are particularly vulnerable to sudden death due to irregular heart rhythms. These could be prevented with medication or implantable defibrillators, which can shock the heart back into rhythm. Right now, Scirica said, it’s difficult to determine who needs them before it’s too late. A majority of patients who have implantable defibrillators never end up needing them. And a majority of people who die of sudden cardiac arrest were not shown to be candidates for a defibrillator using today’s tests.
Finding predictive computational biomarkers
Syed and his colleagues developed new ways to parse through the data to find abnormalities — “computational biomarkers” — that point to defects in the heart muscle and nervous system that evolve over time.
The examined three biomarkers: morphologic variability, the amount of subtle variability in the shape of apparently normal-looking heartbeats over long periods of time; heart rate motifs, specific sequences of changes in heart rate that reflect whether the heart is responding to nervous system signals as it should; and symbolic mismatch, which measures how different a patient’s long-term EKG signal is compared with those of other patients with similar clinical histories.
To prove that patients in the study whose EKG signals had these properties were sicker and more likely to die, the researchers used the signals to pick out who was still alive a year after a heart attack, and who was not. They found that those with at least one of the abnormalities were between two and three times more likely to die within 12 months. And by adding all three of the techniques to doctors’ current assessment tools, they could predict 50 percent more deaths with fewer false positives.
“That translates into thousands or tens of thousands of patients for whom doctors could potentially prescribe an effective preventative treatment based on a more individualized assessment of their risk of complications,” Syed said.
The new techniques use data that is already routinely collected during hospital visits, so putting them into practice would not raise costs or further burden caregivers or patients.
“Our findings demonstrate that low cost, non-invasive, and easily obtained biometric data, such as the ECG, can be used to identify patients at high risk of adverse outcomes. We hope that this work ignites a new paradigm in the field of cardiovascular risk stratification,” said Collin Stultz, a cardiologist and an associate professor in the MIT Department of Electrical Engineering and Computer Science and the Harvard-MIT Division of Health Sciences and Technology. Stultz is also affiliated with CSAIL.
Ref.: Zeeshan Syed, et al., Computationally Generated Cardiac Biomarkers for Risk Stratification After Acute Coronary Syndrome, Science Translational Medicine, 2011; [DOI: 10.1126/scitranslmed.3002557]