Data mining opens the door to predictive neuroscience
April 12, 2012
Ecole Polytechnique Fédérale de Lausanne (EPFL) researchers have discovered rules that relate the genes that a neuron switches on and off to the shape of that neuron, its electrical properties, and its location in the brain.
The discovery, using state-of-the-art computational tools, increases the likelihood that it will be possible to predict much of the fundamental structure and function of the brain without having to measure every aspect of it.
That in turn makes modeling the brain in silico — the goal of the proposed Human Brain Project — a more realistic, less Herculean, prospect.
“It is the door that opens to a world of predictive biology,” says Prof. Henry Markram.
Background
Within a cortical column, the basic processing unit of the mammalian brain, there are roughly 300 different neuronal types. These types are defined both by their anatomical structure and by their electrical properties, and their electrical properties are in turn defined by the combination of ion channels they present — the tiny pores in their cell membranes through which electrical current passes, which make communication between neurons possible.
Scientists would like to be able to predict, based on a minimal set of experimental data, which combination of ion channels a neuron presents. They know that genes are often expressed together, perhaps because two genes share a common promoter (the stretch of DNA that allows a gene to be transcribed and, ultimately, translated into a functioning protein) or because one gene modifies the activity of another. So expression of certain gene combinations is informative about a neuron’s characteristics.
Extracting rules from gene expression patterns and other data
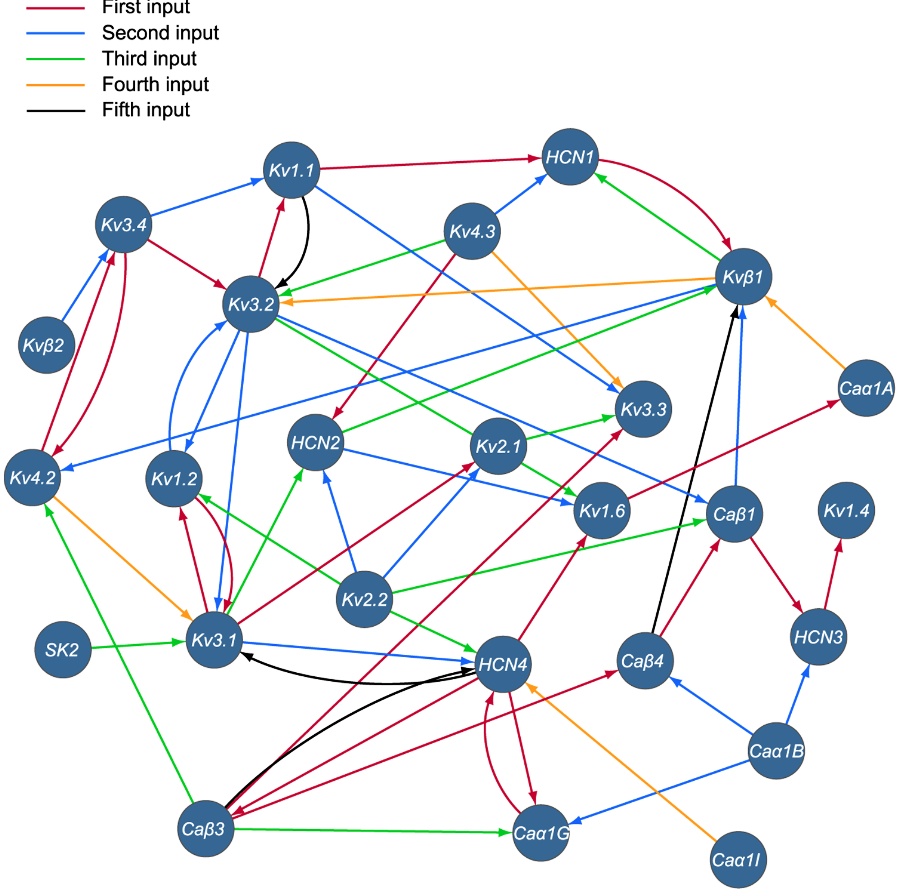
Directed network diagram showing links from input ion channel genes to their corresponding output channels. The edges are colored in red, blue, green, orange, and black based on the predictive value of the input ion channel gene. Red edges have the highest predictive value and represent the genes that were selected as inputs at the first incremental step; black edges have the lowest predictive value and represent the genes that were selected at the last incremental step. (Credit: Georges Khazen/PLoS ONE)
The researchers hypothesised that they could extract rules from gene expression patterns to predict the characteristics of neurons.
They took a dataset that Markram and others had collected a few years ago, in which they recorded the expression of 26 genes encoding ion channels in different neuronal types from the rat brain. They also had data classifying those types according to a neuron’s morphology (form), its electrophysiological properties, and its position within the six anatomically distinct layers of the cortex.
They found that based on the classification data alone, they could predict those previously measured ion channel patterns with 78 per cent accuracy. And when they added in a subset of data about the ion channels to the classification data, as input to their data-mining program, they were able to boost that accuracy to 87 per cent for the more commonly occurring neuronal types.
Predicting all ion channels for a neuron
“This shows that it is possible to mine rules from a subset of data and use them to complete the dataset informatically,” says one of the study’s authors, Felix Schürmann. “Using the methods we have developed, it may not be necessary to measure every single aspect of the behavior you’re interested in.”
Once the rules have been validated in similar but independently collected datasets, for example, they could be used to predict the entire complement of ion channels presented by a given neuron, based simply on data about that neuron’s morphology, its electrical behaviour and a few key genes that it expresses.
Researchers could also use such rules to explore the roles of different genes in regulating transcription processes. And importantly, if rules exist for ion channels, they are also likely to exist for other aspects of brain organization.
For example, the researchers believe it will be possible to predict where synapses are likely to form in neuronal networks, based on information about the ratio of neuronal types in that network. Knowledge of such rules could usher in a new era of predictive biology, and accelerate progress towards understanding and modeling the brain.
Ref.: Georges Khazen, Sean L. Hill, Felix Schürmann, Henry Markram. Combinatorial Expression Rules of Ion Channel Genes in Juvenile Rat (Rattus norvegicus) Neocortical Neurons. PLoS ONE, 2012 [DOI: 10.1371/journal.pone.0034786]