Deep learning-based bionic hand grasps objects automatically
May 5, 2017
British biomedical engineers have developed a new generation of intelligent prosthetic limbs that allows the wearer to reach for objects automatically, without thinking — just like a real hand.
The hand’s camera takes a picture of the object in front of it, assesses its shape and size, picks the most appropriate grasp, and triggers a series of movements in the hand — all within milliseconds.
The research finding was published Wednesday May 3 in an open-access paper in the Journal of Neural Engineering.
A deep learning-based artificial vision and grasp system
Biomedical engineers at Newcastle University and associates developed a convolutional neural network (CNN), trained it with images of more than 500 graspable objects, and taught it to recognize the grip needed for different types of objects.
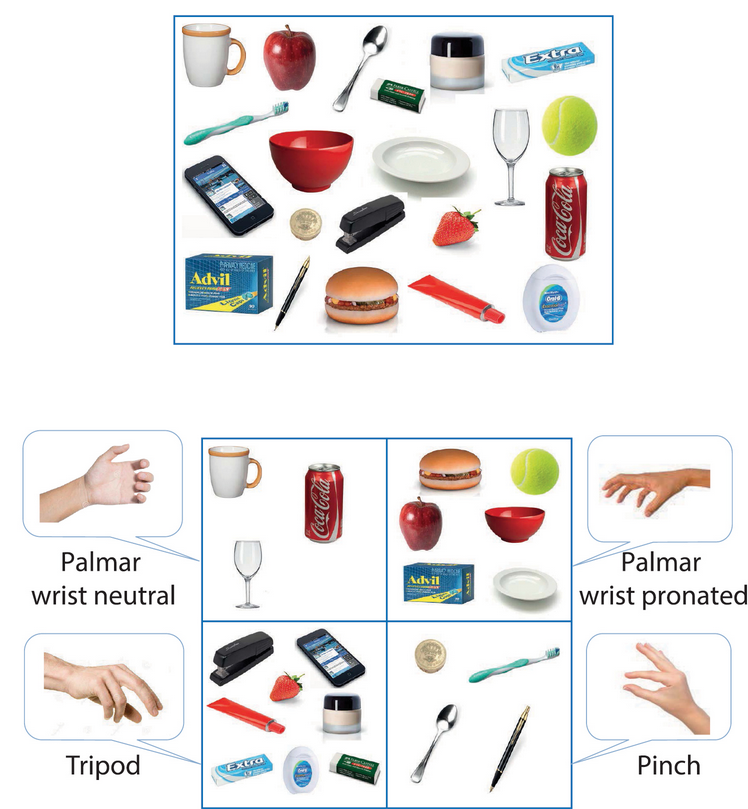
Object recognition (top) vs. grasp recognition (bottom) (credit: Ghazal Ghazaei/Journal of Neural Engineering)
Grouping objects by size, shape and orientation, according to the type of grasp that would be needed to pick them up, the team programmed the hand to perform four different grasps: palm wrist neutral (such as when you pick up a cup); palm wrist pronated (such as picking up the TV remote); tripod (thumb and two fingers), and pinch (thumb and first finger).
“We would show the computer a picture of, for example, a stick,” explains lead author Ghazal Ghazae. “But not just one picture; many images of the same stick from different angles and orientations, even in different light and against different backgrounds, and eventually the computer learns what grasp it needs to pick that stick up.”
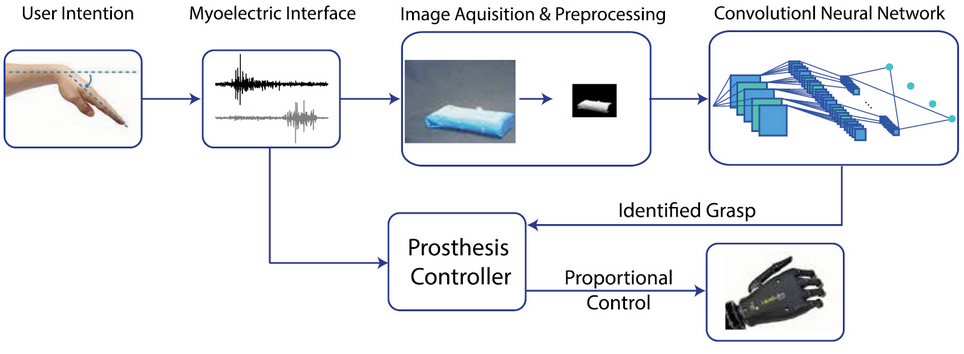
A block diagram representation of the method (credit: Ghazal Ghazaei/Journal of Neural Engineering)
Current prosthetic hands are controlled directly via the user’s myoelectric signals (electrical activity of the muscles recorded from the skin surface of the stump). That takes learning, practice, concentration and, crucially, time.
A small number of amputees have already trialed the new technology. After training, subjects successfully picked up and moved the target objects with an overall success of up to 88%. Now the Newcastle University team is working with experts at Newcastle upon Tyne Hospitals NHS Foundation Trust to offer the “hands with eyes” to patients at Newcastle’s Freeman Hospital.
A future bionic hand
The work is part of a larger research project to develop a bionic hand that can sense pressure and temperature and transmit the information back to the brain.
Led by Newcastle University and involving experts from the universities of Leeds, Essex, Keele, Southampton and Imperial College London, the aim is to develop novel electronic devices that connect neural networks to the forearm to allow two-way communications with the brain.
The research is funded by the Engineering and Physical Sciences Research Council (EPSRC).
Abstract of Deep learning-based artificial vision for grasp classification in myoelectric hands
Objective. Computer vision-based assistive technology solutions can revolutionise the quality of care for people with sensorimotor disorders. The goal of this work was to enable trans-radial amputees to use a simple, yet efficient, computer vision system to grasp and move common household objects with a two-channel myoelectric prosthetic hand. Approach. We developed a deep learning-based artificial vision system to augment the grasp functionality of a commercial prosthesis. Our main conceptual novelty is that we classify objects with regards to the grasp pattern without explicitly identifying them or measuring their dimensions. A convolutional neural network (CNN) structure was trained with images of over 500 graspable objects. For each object, 72 images, at intervals, were available. Objects were categorised into four grasp classes, namely: pinch, tripod, palmar wrist neutral and palmar wrist pronated. The CNN setting was first tuned and tested offline and then in realtime with objects or object views that were not included in the training set. Main results. The classification accuracy in the offline tests reached
for the seen and
for the novel objects; reflecting the generalisability of grasp classification. We then implemented the proposed framework in realtime on a standard laptop computer and achieved an overall score of
in classifying a set of novel as well as seen but randomly-rotated objects. Finally, the system was tested with two trans-radial amputee volunteers controlling an i-limb UltraTM prosthetic hand and a motion controlTM prosthetic wrist; augmented with a webcam. After training, subjects successfully picked up and moved the target objects with an overall success of up to
. In addition, we show that with training, subjects’ performance improved in terms of time required to accomplish a block of 24 trials despite a decreasing level of visual feedback. Significance. The proposed design constitutes a substantial conceptual improvement for the control of multi-functional prosthetic hands. We show for the first time that deep-learning based computer vision systems can enhance the grip functionality of myoelectric hands considerably.