Deep neural network program recognizes sketches more accurately than a human
July 21, 2015
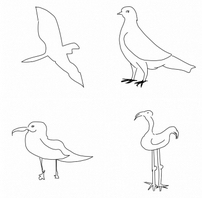
The Sketch-a-Net program successfully identified a seagull, pigeon, flying bird and standing bird better than humans (credit: QMUL, Mathias Eitz, James Hays and Marc Alexa)
The first computer program that can recognize hand-drawn sketches better than humans has been developed by researchers from Queen Mary University of London.
Known as Sketch-a-Net, the program correctly identified the subject of sketches 74.9 per cent of the time compared to humans that only managed a success rate of 73.1 per cent.
As sketching becomes more relevant with the increase in the use of touchscreens, it could lead to new ways to interact with computers. Touchscreens could understand what you are drawing enabling you to retrieve a specific image by drawing it with your fingers, which is more natural than keyword searches for finding items such as furniture or fashion accessories.
The improvement could also aid police forensics when an artist’s impression of a criminal needs to be matched to a mugshot or CCTV database.
The research also showed that the program performed better at determining finer details in sketches. For example, it was able to successfully distinguish “seagull,” “flying-bird,” “standing-bird” and “pigeon” with 42.5 per cent accuracy compared to humans, who only achieved 24.8 per cent.
Sketch-a-Net is a “deep neural network” program, designed to emulate the processing of the human brain. It is particularly successful because it accommodates the unique characteristics of sketches, particularly the order the strokes were drawn. This was information that was previously ignored but is especially important for understanding drawings on touchscreens.
Abstract of Sketch-a-Net that Beats Humans
We propose a multi-scale multi-channel deep neural network framework that, for the first time, yields sketch recognition performance surpassing that of humans. Our superior performance is a result of explicitly embedding the unique characteristics of sketches in our model: (i) a network architecture designed for sketch rather than natural photo statistics, (ii) a multi-channel generalisation that encodes sequential ordering in the sketching process, and (iii) a multi-scale network ensemble with joint Bayesian fusion that accounts for the different levels of abstraction exhibited in free-hand sketches. We show that state-of-the-art deep networks specifically engineered for photos of natural objects fail to perform well on sketch recognition, regardless whether they are trained using photo or sketch. Our network on the other hand not only delivers the best performance on the largest human sketch dataset to date, but also is small in size making efficient training possible using just CPUs.