First robotic legs to fully model biologically accurate walking
July 6, 2012
University of Arizona researchers have produced a robotic set of legs that they believe is the first to fully model walking in a biologically accurate manner.
The neural architecture, musculoskeletal architecture, and sensory feedback pathways in humans have been simplified and built into the robot, giving it a remarkably human-like walking gait that can be viewed in the video.
The biological accuracy of this robot, presented today (Friday July 6), in IOP Publishing’s Journal of Neural Engineering (open access), has allowed the researchers to investigate the processes underlying walking in humans and may bolster theories of how babies learn to walk, as well as helping to understand how spinal-cord-injury patients can recover the ability to walk.
Central pattern generator
A key component of the human walking system is the central pattern generator (CPG). The CPG is a neural network in the lumbar region of the spinal cord that generates rhythmic muscle signals. The CPG produces and then controls these signals by gathering information from different parts of the body that are responding to the environment. This is what allows people to walk without needing to think about it.
The simplest form of a CPG is a half-center, which consists of just two neurons that fire signals alternatively, producing a rhythm. The robot contains an artificial half-center as well as sensors that deliver information back to the half-center, including load sensors that sense force in the limb when the leg is pressed against a stepping surface.
“Interestingly, we were able to produce a walking gait, without balance, which mimicked human walking with only a simple half-center controlling the hips and a set of reflex responses controlling the lower limb,” said Dr. Theresa Klein, co-author of the study.
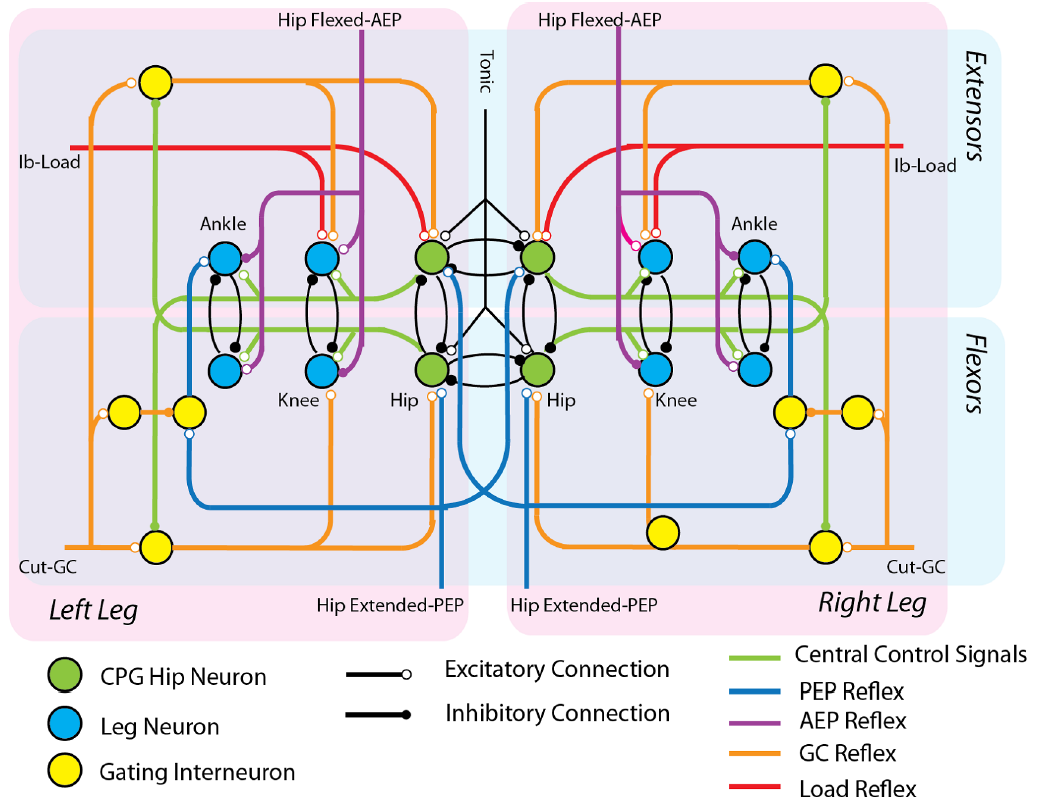
Neural Architecture of Single-level Simplified CPG model. Four central neurons control hip flexion and extension. Environmental feedback from ground contact sensors adapt lower leg motion in the knee and ankle. Ground contact (GC) and load (Load) sensors feed back to knee and hip extension. Anterior extreme position (AEP) angle sensors extend the knee to prepare for foot touchdown, while posterior extreme position (PEP) sensors extend the ankle during toe off, and trigger flexion at the hip and knee. And weak descending connections link the four central neurons to the knees and ankles. (Credit: Theresa J Klein and M. Anthony Lewis /University of Arizona)
The researchers hypothesize that babies start off with a simple half-center, similar to the one developed in this robot, and over time they “learn” a network for a more complex walking pattern. This could explain why babies have been seen to exhibit a simple walking pattern when placed on a treadmill even before they have learned to walk — a simple half-center is already in place.
“This underlying network may also form the core of the CPG and may explain how people with spinal cord injuries can regain walking ability if properly stimulated in the months after the injury,” said Klein.