Helping researchers cope with the medical literature knowledge explosion
August 27, 2014
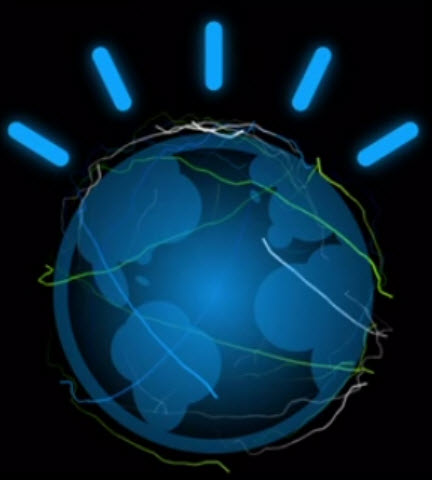
(Credit: IBM)
Computational biologists at Baylor College of Medicine and analytics experts at IBM research are developing a powerful new tool called the Knowledge Integration Toolkit (KnIT) that promises to help research scientists deal with the more than 50 million scientific papers available in public databases — with a new one publishing nearly every 30 seconds.
The goal: allow researchers pursuing new scientific studies to mine all available medical literature and formulate hypotheses that promise the greatest reward.
In a case study using KnIT, researchers predicted the existence of proteins that modify p53 (an important tumor suppressor protein). These proteins were later found to do just that*.
Details from the study, “Automated hypothesis generation based on mining scientific literature,” were published online Monday in the Association for Computing Machinery‘s digital library.
“Even if a scientist reads five papers a day, it could take nearly 38 years to completely understand all of the research already available today on this protein.” — Olivier Lichtarge
Olivier Lichtarge, PhD, director of the Center of Computational and Integrative Biomedical Research at Baylor and the principal investigator on the study, will discuss details of the study in a presentation today (Wednesday Aug. 27) at the 20th annual Association for Computing Machinery’s Special Interest Group on Knowledge Discovery and Data Mining conference in New York City, the premier data-mining conference.
How to access information from 70,000 papers
“On average, a scientist might read between one and five research papers on a good day,” said Lichtarge, also a professor of molecular and human genetics, biochemistry and molecular biology at Baylor. “But to put this in perspective with p53, there are over 70,000 papers published on this protein.
“Even if a scientist reads five papers a day, it could take nearly 38 years to completely understand all of the research already available today on this protein.”
Scientists formulate hypotheses based on what they read and know, but because there is so little that they can actually read, hypotheses can be biased, Lichtarge notes. “A computer certainly may not reason as well as a scientist, but it can, logically and objectively, contribute greatly when applied to our entire body of knowledge.”
Watson to accelerate understanding of the biology underlying diseases
Working with colleagues at IBM led by Scott Spangler, principal data scientist at IBM, the team took advantage of existing text mining capabilities, such as those used by IBM’s Watson technology.
“Our hope is that scientists and researchers will be able to use Watson’s cognitive capabilities to accelerate the understanding of biology underlying diseases,” said Spangler. “Better understanding the biology of diseases can eventually lead to better treatments for some of the most complex and challenging diseases, like cancer.”
KnIT represents the knowledge explicitly in a network that can be queried, and then allows for further attempts to use these data to generate new reasonable and testable hypotheses that can be used to help direct laboratory studies.
“Our long-term hope is to systematically extract knowledge directly from the totality of the public medical literature. For this we need technological advances to read text, extract facts from every sentence and to integrate this information into a network that describes the relationship between all of the objects and entities discussed in the literature,” said Lichtarge.
“This first study is promising, because it suggests a proof of principle for a small step towards this type of knowledge discovery. With more research, we hope to get closer to clinical and therapeutic applications.”
Most of the funding for this work was provided by the McNair Medical Institute of the Robert and Janice McNair Foundation and the Defense Advanced Research Projects Agency. Additional funding was provided by the National Science Foundation, and National Institutes of Health, and was supported in part by the IBM Accelerated Discovery Lab. Scientists at the University of Texas M.D. Anderson Cancer Center where also involved in the study.
* In the first test using KnIT, the team sought to identify new protein kinases that phosphorylate (or turn on) the protein tumor suppressor p53. There are over 500 known human kinases and 10s of thousands of possible proteins they can target. Thirty-three are currently known to modify p53.
In the study, the team used KnIT to mine the medical literature up to 2003 when only half of the 33 phosphorylating protein kinases had been discovered.
Using KnIT, 74 kinases were extracted as potential modifiers. Of these, prior to 2003, 10 were known to phosphorylate p53, nine were discovered at a later date. Of the 10 already known, KnIT accounted for them in reasoning as well as ranking the likelihood that the other 64 kinases targeted p53. Of the nine found nearly a decade later, KnIT accurately predicted seven.
“This study showed that in a very narrow field of study regarding p53, we can, in fact, suggest new relationships and new functions associated with p53, which can later be directly validated in the laboratory,” said Lichtarge, who holds The Cullen Foundation Endowed Chair at Baylor.
The remaining kinases identified in the case study, but not previously identified in real time, may be further studied in the laboratory, he said.
Abstract of Proceedings of the 20th ACM SIGKDD international conference on Knowledge discovery and data mining paper
Keeping up with the ever-expanding flow of data and publications is untenable and poses a fundamental bottleneck to scientific progress. Current search technologies typically find many relevant documents, but they do not extract and organize the information content of these documents or suggest new scientific hypotheses based on this organized content. We present an initial case study on KnIT, a prototype system that mines the information contained in the scientific literature, represents it explicitly in a queriable network, and then further reasons upon these data to generate novel and experimentally testable hypotheses. KnIT combines entity detection with neighbor-text feature analysis and with graph-based diffusion of information to identify potential new properties of entities that are strongly implied by existing relationships. We discuss a successful application of our approach that mines the published literature to identify new protein kinases that phosphorylate the protein tumor suppressor p53. Retrospective analysis demonstrates the accuracy of this approach and ongoing laboratory experiments suggest that kinases identified by our system may indeed phosphorylate p53. These results establish proof of principle for automated hypothesis generation and discovery based on text mining of the scientific literature.