Machine learning accelerates the discovery of new materials
May 9, 2016
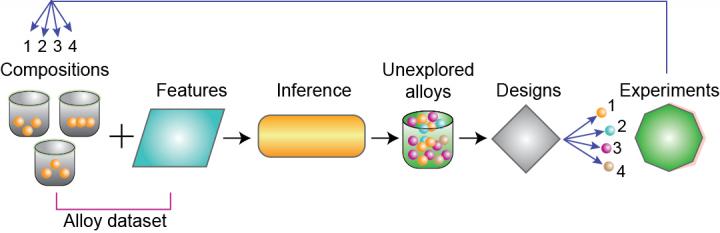
Feedback from experiments: augmented dataset with four new alloys (credit: Los Alamos National Laboratory)
Scientists at Los Alamos National Laboratory and the State Key Laboratory for Mechanical Behavior of Materials in China have used a combination of machine learning, supercomputers, and experiments to speed up discovery of new materials with desired properties.
The idea is to replace traditional trial-and-error materials research, which is guided only by intuition (and errors). With increasing chemical complexity, the possible combinations have become too large for those trial-and-error approaches to be practical.
The scientists focused their initial research on improving nickel-titanium (nitinol) shape-memory alloys (materials that can recover their original shape at a specific temperature after being bent). But the strategy can be used for any materials class (polymers, ceramics, or nanomaterials) or target properties (e.g., dielectric response, piezoelectric coefficients, and band gaps).
Cutting time and cost of creating new materials
“What we’ve done is show that, starting with a relatively small data set of well-controlled experiments, it is possible to iteratively guide subsequent experiments toward finding the material with the desired target,” said principal investigator Turab Lookman, a physicist and materials scientist in the Physics of Condensed Matter and Complex Systems group at Los Alamos. “The goal is to cut in half the time and cost of bringing materials to market,” he said.
The impetus for the research was a 2013 announcement by the Obama Administration and academic and industry partners of the Materials Genome Initiative, a public-private endeavor that aims to cut in half the time it takes to develop novel materials that can fuel advanced manufacturing and bolster the American economy. This new study is one of the first to demonstrate how an informatics framework can do that.*
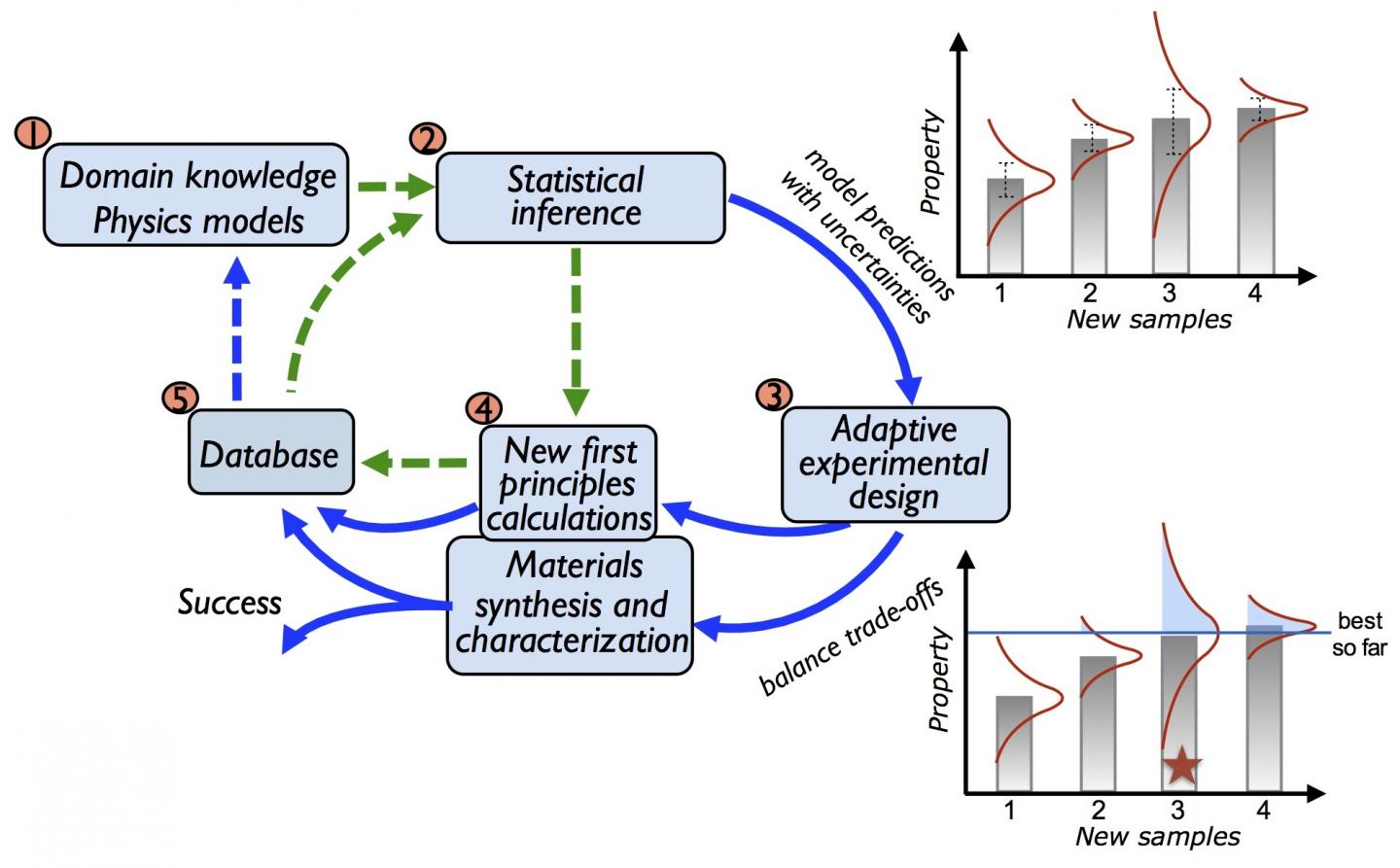
Adaptive design framework (credit: Los Alamos National Laboratory)
Although the new research focused on the chemical exploration space, it can be readily adapted to optimize processing conditions when there are many “tuning knobs” controlling a figure of merit, as in advanced manufacturing applications, or to optimize multiple properties, such as (in the case of the nickel-titanium-based alloy) low dissipation and a transition temperature several degrees above room temperature.
The research was published in an open-access paper in Nature Communications. The Laboratory Directed Research and Development (LDRD) program at Los Alamos funded the work and the lab provided institutional computing resources.
* Much of the effort in the field has centered on generating and screening databases typically formed by running thousands of quantum mechanical calculations. However, the interplay of structural, chemical and microstructural degrees of freedom introduces enormous complexity, especially if defects, solid solutions, and multi-component compounds are involved, which the current state-of-the-art tools are not yet designed to handle. Moreover, few studies include any feedback to experiments or incorporate uncertainties. This becomes important when experiments or calculations are costly and time-consuming.
Abstract of Accelerated search for materials with targeted properties by adaptive design
Finding new materials with targeted properties has traditionally been guided by intuition, and trial and error. With increasing chemical complexity, the combinatorial possibilities are too large for an Edisonian approach to be practical. Here we show how an adaptive design strategy, tightly coupled with experiments, can accelerate the discovery process by sequentially identifying the next experiments or calculations, to effectively navigate the complex search space. Our strategy uses inference and global optimization to balance the trade-off between exploitation and exploration of the search space. We demonstrate this by finding very low thermal hysteresis (ΔT) NiTi-based shape memory alloys, with Ti50.0Ni46.7Cu0.8Fe2.3Pd0.2 possessing the smallest ΔT (1.84 K). We synthesize and characterize 36 predicted compositions (9 feedback loops) from a potential space of ~800,000 compositions. Of these, 14 had smaller ΔT than any of the 22 in the original data set.