Planarian regeneration model discovered by AI algorithm
June 4, 2015
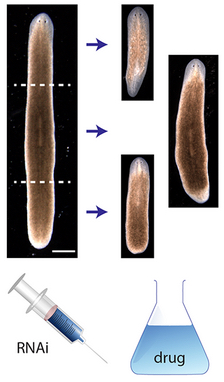
Head-trunk-tail planarian regeneration results from experiments (credit: Daniel Lobo and Michael Levin/PLOS Computational Biology)
An artificial intelligence system has for the first time reverse-engineered the regeneration mechanism of planaria — the small worms whose extraordinary power to regrow body parts has made them a research model in human regenerative medicine.
The discovery by Tufts University biologists presents the first model of regeneration discovered by a non-human intelligence and the first comprehensive model of planarian regeneration, which had eluded human scientists for more than 100 years. The work, published in the June 4 issue of PLOS Computational Biology (open access), demonstrates how “robot science” can help human scientists in the future.
To bioengineer complex organs, scientists need to understand the mechanisms by which those shapes are normally produced by the living organism.
However, there’s a significant knowledge gap between the molecular genetic components needed to produce a particular organism shape and understanding how to generate that particular complex shape in the correct size, shape and orientation, said the paper’s senior author, Michael Levin, Ph.D., Vannevar Bush professor of biology and director of the Tufts Center for Regenerative and Developmental Biology.
“Most regenerative models today derived from genetic experiments are arrow diagrams, showing which gene regulates which other gene. That’s fine, but it doesn’t tell you what the ultimate shape will be. You cannot tell if the outcome of many genetic pathway models will look like a tree, an octopus or a human,” said Levin.
“Most models show some necessary components for the process to happen, but not what dynamics are sufficient to produce the shape, step by step. What we need are algorithmic or constructive models, which you could follow precisely and there would be no mystery or uncertainty. You follow the recipe and out comes the shape.”
Such models are required to know what triggers could be applied to such a system to cause regeneration of particular components, or other desired changes in shape. However, no such tools yet exist for mining the fast-growing mountain of published experimental data in regeneration and developmental biology, said the paper’s first author, Daniel Lobo, Ph.D., post-doctoral fellow in the Levin lab.
An evolutionary computation algorithm
To address this challenge, Lobo and Levin developed an algorithm that could be used to produce regulatory networks able to “evolve” to accurately predict the results of published laboratory experiments that the researchers entered into a database.
“Our goal was to identify a regulatory network that could be executed in every cell in a virtual worm so that the head-tail patterning outcomes of simulated experiments would match the published data,” Lobo said.
The algorithm generated networks by randomly combining previous networks and performing random changes, additions and deletions. Each candidate network was tested in a virtual worm, under simulated experiments. The algorithm compared the resulting shape from the simulation with real published data in the database.
As evolution proceeded, gradually the new networks could explain more experiments in the database comprising most of the known planarian experimental literature regarding head vs. tail regeneration.
Regenerative model discovered by AI
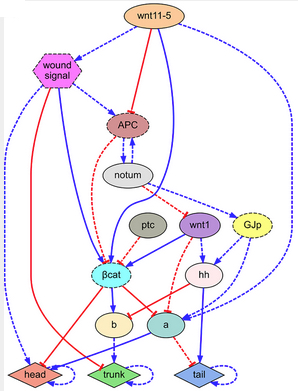
Regulatory network found by the automated system, explaining the combined phenotypic (forms and characteristics) experimental data of the key publications of head-trunk-tail planarian regeneration (credit: Daniel Lobo and Michael Levin/PLOS Computational Biology)
The researchers ultimately applied the algorithm to a combined experimental dataset of 16 key planarian regeneration experiments to determine if the approach could identify a comprehensive regulatory network of planarian generation.
After 42 hours, the algorithm returned the discovered regulatory network, which correctly predicted all 16 experiments in the dataset. The network comprised seven known regulatory molecules as well as two proteins that had not yet been identified in existing papers on planarian regeneration.
“This represents the most comprehensive model of planarian regeneration found to date. It is the only known model that mechanistically explains head-tail polarity determination in planaria under many different functional experiments and is the first regenerative model discovered by artificial intelligence,” said Levin.
The paper represents a successful application of the growing field of “robot science.”
“While the artificial intelligence in this project did have to do a whole lot of computations, the outcome is a theory of what the worm is doing, and coming up with theories of what’s going on in nature is pretty much the most creative, intuitive aspect of the scientist’s job,” Levin said.
“One of the most remarkable aspects of the project was that the model it found was not a hopelessly-tangled network that no human could actually understand, but a reasonably simple model that people can readily comprehend. All this suggests to me that artificial intelligence can help with every aspect of science, not only data mining, but also inference of meaning of the data.”
This work was supported with funding from the National Science Foundation, National Institutes of Health, USAMRMC, and the Mathers Foundation.