Researchers build brain-machine interface to control prosthetic hand
March 31, 2015
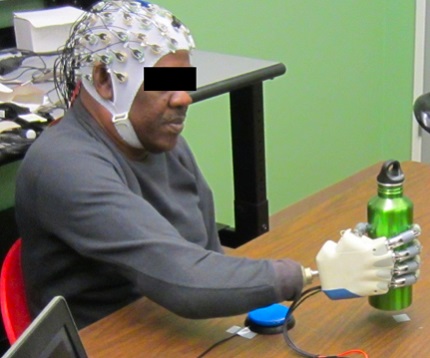
New UH research has demonstrated that an amputee can grasp with a bionic hand, powered only by his thoughts (credit: University of Houston)
A research team from the University of Houston has created an algorithm that allowed a man to grasp a bottle and other objects with a prosthetic hand, controlled only by his thoughts.
The technique, demonstrated with a 56-year-old man whose right hand had been amputated, uses non-invasive brain monitoring, capturing brain activity to determine what parts of the brain are involved in grasping an object.
With that information, researchers created a computer program, or brain-machine interface (BMI), that harnessed the subject’s intentions and allowed him to successfully grasp objects, including a water bottle and a credit card. The subject grasped the selected objects 80 percent of the time using a high-tech bionic hand fitted to the amputee’s stump.
Previous studies involving either surgically implanted electrodes or myoelectric control, which relies upon electrical signals from muscles in the arm, have shown similar success rates, according to the researchers. But the non-invasive method offers several advantages, says Jose Luis Contreras-Vidal, a neuroscientist and engineer at UH. It avoids the risks of surgically implanting electrodes by measuring brain activity via scalp electroencephalogram, or EEG. And myoelectric systems aren’t an option for all people, because they require that neural activity from muscles relevant to hand grasping remain intact.
The results of the study were published March 30 in Frontiers in Neuroscience.
The work, funded by the National Science Foundation, demonstrates for the first time EEG-based BMI control of a multi-fingered prosthetic hand for grasping by an amputee. It also could lead to the development of better prosthetics, Contreras-Vidal said.
Using non-invasive EEG also offers a new understanding of the neuroscience of grasping and will be applicable to rehabilitation for other types of injuries, including stroke and spinal cord injury, the researchers said.
T he study subjects were tested using a 64-channel active EEG, with electrodes attached to the scalp to capture brain activity. Brain activity was recorded in multiple areas, including the motor cortex and areas known to be used in action observation and decision-making, and occurred between 50 milliseconds and 90 milliseconds before the hand began to grasp.
That provided evidence that the brain predicted the movement, rather than reflecting it, he said.
“Current upper limb neuroprosthetics restore some degree of functional ability, but fail to approach the ease of use and dexterity of the natural hand, particularly for grasping movements,” the researchers wrote, noting that work with invasive cortical electrodes has been shown to allow some hand control but not at the level necessary for all daily activities.
“Further, the inherent risks associated with surgery required to implant electrodes, along with the long-term stability of recorded signals, is of concern. Here we show that it is feasible to extract detailed information on intended grasping movements to various objects in a natural, intuitive manner, from a plurality of scalp EEG signals.”
Until now, this was thought to be possible only with brain signals acquired invasively inside or on the surface of the brain.
Researchers first recorded brain activity and hand movement in the able-bodied volunteers as they picked up five objects, each chosen to illustrate a different type of grasp: a soda can, a compact disc, a credit card, a small coin and a screwdriver. The recorded data were used to create decoders of neural activity into motor signals, which successfully reconstructed the grasping movements.
They then fitted the amputee subject with a computer-controlled neuroprosthetic hand and told him to observe and imagine himself controlling the hand as it moved and grasped the objects.
The subject’s EEG data, along with information about prosthetic hand movements gleaned from the able-bodied volunteers, were used to build the algorithm.
Contreras-Vidal said additional practice, along with refining the algorithm, could increase the success rate to 100 percent.
Abstract of Global cortical activity predicts shape of hand during grasping
Recent studies show that the amplitude of cortical field potentials is modulated in the time domain by grasping kinematics. However, it is unknown if these low frequency modulations persist and contain enough information to decode grasp kinematics in macro-scale activity measured at the scalp via electroencephalography (EEG). Further, it is unclear as to whether joint angle velocities or movement synergies are the optimal kinematics spaces to decode. In this offline decoding study, we infer from human EEG, hand joint angular velocities as well as synergistic trajectories as subjects perform natural reach-to-grasp movements. Decoding accuracy, measured as the correlation coefficient (r) between the predicted and actual movement kinematics, was r = 0.49 ± 0.02 across fifteen hand joints. Across the first three kinematic synergies, decoding accuracies were r = 0.59 ± 0.04, 0.47 ± 0.06 and 0.32 ± 0.05. The spatial-temporal pattern of EEG channel recruitment showed early involvement of contralateral frontal-central scalp areas followed by later activation of central electrodes over primary sensorimotor cortical areas. Information content in EEG about the grasp type peaked at 250 ms after movement onset. The high decoding accuracies in this study are significant not only as evidence for time-domain modulation in macro-scale brain activity, but for the field of brain-machine interfaces as well. Our decoding strategy, which harnesses the neural ‘symphony’ as opposed to local members of the neural ensemble (as in intracranial approaches), may provide a means of extracting information about motor intent for grasping without the need for penetrating electrodes and suggests that it may be soon possible to develop non-invasive neural interfaces for the control of prosthetic limbs.