Using machine learning to rationally design future electronics materials
March 14, 2016
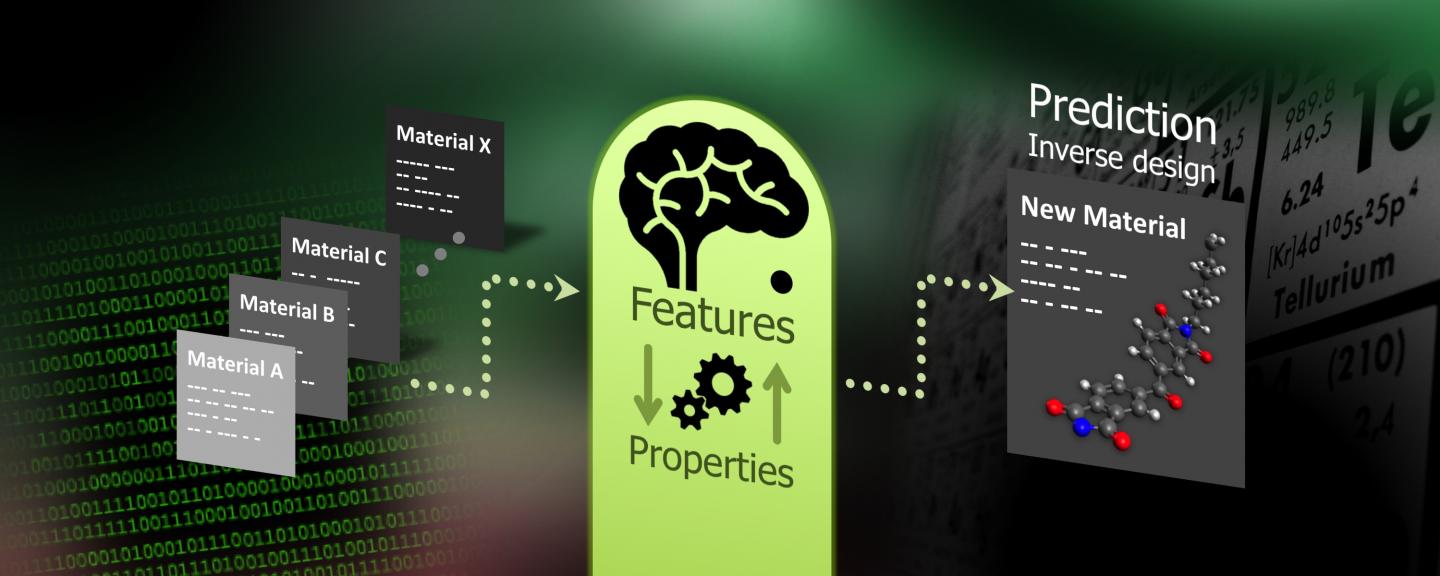
A schematic diagram of machine learning for materials discovery (credit: Chiho Kim, Ramprasad Lab, UConn)
Replacing inefficient experimentation, UConn researchers have used machine learning to systematically scan millions of theoretical compounds for qualities that would make better materials for solar cells, fibers, and computer chips.
Led by UConn materials scientist Ramamurthy ‘Rampi’ Ramprasad, the researchers set out to determine which polymer atomic configurations make a given polymer a good electrical conductor or insulator, for example.
A polymer is a large molecule made of many repeating building blocks. The most familiar example is plastics. What controls a polymer’s properties is mainly how the atoms in the polymer connect to each other. Polymers can also have diverse electronic properties. For example, they can be very good insulators or good conductors. And what controls all these properties is mainly how the atoms in the polymer connect to each other.
But with at least 95 stable elements, the number of possible combinations is astronomical. So they pared down the problem to a manageable subset. Many polymers are made of building blocks containing just a few atoms. They look like this:
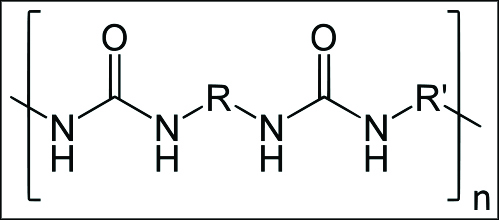
Polyurea, a common plastic. In this diagram, N is nitrogen, H hydrogen, and O oxygen. R stands in for any number of chemicals that could slightly alter the polymer, but the repeating NH-O-NH-O is the basic structure. Most polymers look like that, made of carbon (C), H, N and O, with a few other elements thrown in occasionally. (credit: Yikrazuul/public domain)
For their project, Ramprasad’s group looked at polymers made of just seven building blocks: CH2, C6H4, CO, O, NH, CS, and C4H2S. These are found in common plastics such as polyethylene, polyesters, and polyureas. An enormous variety of polymers could theoretically be constructed using just these building blocks; Ramprasad’s group decided at first to analyze just 283, each composed of a repeated four-block unit.
They started from basic quantum mechanics, and calculated the three-dimensional atomic and electronic structures of each of those 283 four-block polymers (calculating the position of every electron and atom in a molecule with more than two atoms takes a powerful computer a significant chunk of time, which is why they did it for only 283 molecules).
Calculating key electronic properties
Once they had the three-dimensional structures, they could calculate what they really wanted to know: each polymer’s properties.
- Ramprasad’s group calculated the band gap, which is the amount of energy it takes for an electron in the polymer to break free of its home atom and travel around the material; and the dielectric constant, which is a measure of the effect an electric field can have on the polymer. These properties translate to how much electric energy each polymer can store in itself.
- They then defined each polymer as a string of numbers, a sort of numerical fingerprint. Since there are seven possible building blocks, there are seven possible numbers, each indicating how many of each block type are contained in that polymer.
- But a simple number string like that doesn’t give enough information about the polymer’s structure, so they added a second string of numbers that tell how many pairs there are of each combination of building blocks, such as NH-O or C6H4-CS.
- Then they added a third string that described how many triples, like NH-O-CH2, there were. They arranged these strings as a three-dimensional matrix, which is a convenient way to describe such strings of numbers in a computer.
- Then they let the computer go to work. Using the library of 283 polymers they had laboriously calculated using quantum mechanics, the machine compared each polymer’s numerical fingerprint to its band gap and dielectric constant, and gradually ‘learned’ which building block combinations were associated with which properties. It could even map those properties onto a two-dimensional matrix of the polymer building blocks.
- Once the machine learned which atomic building block combinations gave which properties, it could accurately evaluate the band gap and dielectric constant for any polymer made of any combination of those seven building blocks, using just the numerical fingerprint of its structure.
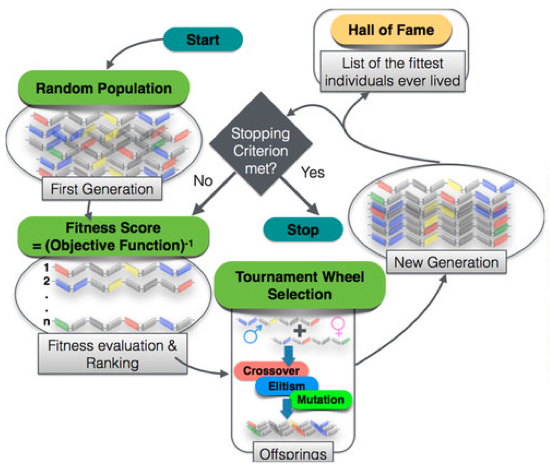
Flow chart of the steps involved in the genetic algorithm (GA) approach, leading to direct design of polymers (credit: Arun Mannodi-Kanakkithodi et al/Scientific Reports)
Validating predictions
Many of the predictions of quantum mechanics and the machine learning scheme have been validated by Ramprasad’s UConn collaborators, who actually made several of the novel polymers and tested their properties.
The group published a paper on their polymer work in an open-access paper in Scientific Reports on Feb. 15; and another paper that utilizes machine learning in a different manner, namely, to discover laws that govern dielectric breakdown of insulators, will be published in a forthcoming issue of Chemistry of Materials.
You can see the predicted properties of every polymer Ramprasad’s group has evaluated in their online data vault, Khazana, which also provides their machine learning apps to predict polymer properties on the fly. They are also uploading data and the machine learning tools from their Chemistry of Materials work, and from an additional recent article published in Scientific Reports on Jan. 19 on predicting the band gap of perovskites, inorganic compounds used in solar cells, lasers, and light-emitting diodes.
Ramprasad’s work is aligned with a larger U.S. White House initiative called the Materials Genome Initiative. Much of Ramprasad’s work described here was funded by grants from the Office of Naval Research, as well as from the U.S. Department of Energy.
Abstract of Machine Learning Strategy for Accelerated Design of Polymer Dielectrics
The ability to efficiently design new and advanced dielectric polymers is hampered by the lack of sufficient, reliable data on wide polymer chemical spaces, and the difficulty of generating such data given time and computational/experimental constraints. Here, we address the issue of accelerating polymer dielectrics design by extracting learning models from data generated by accurate state-of-the-art first principles computations for polymers occupying an important part of the chemical subspace. The polymers are ‘fingerprinted’ as simple, easily attainable numerical representations, which are mapped to the properties of interest using a machine learning algorithm to develop an on-demand property prediction model. Further, a genetic algorithm is utilised to optimise polymer constituent blocks in an evolutionary manner, thus directly leading to the design of polymers with given target properties. While this philosophy of learning to make instant predictions and design is demonstrated here for the example of polymer dielectrics, it is equally applicable to other classes of materials as well.