Why evolution may be intelligent, based on deep learning
January 11, 2016
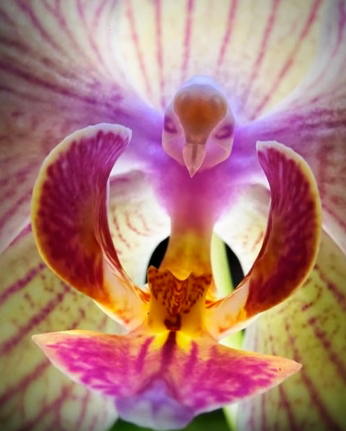
Moth Orchid flower (credit: Christian Kneidinger)
A computer scientist and biologist propose to unify the theory of evolution with learning theories to explain the “amazing, apparently intelligent designs that evolution produces.”
The scientists — University of Southampton School of Electronics and Computer Science professor Richard Watson* and Eötvös Loránd University (Budapest) professor of biology Eörs Szathmáry* — say they’ve found that it’s possible for evolution to exhibit some of the same intelligent behaviors as learning systems — including neural networks.
Writing in an opinion paper published in the journal Trends in Ecology and Evolution, they use “formal analogies” and transfer specific models and results between the two theories in an attempt to solve several evolutionary puzzles.
The authors cite work by Pavlicev and colleagues** showing that selection on relational alleles (gene variants) increases phenotypic (organism trait) correlation if the traits are selected together and decreases correlation if they are selected antagonistically, which is characteristic of Hebbian learning, they note.
“This simple step from evolving traits to evolving correlations between traits is crucial; it moves the object of natural selection from fit phenotypes (which ultimately removes phenotypic variability altogether) to the control of phenotypic variability,” the researchers say.
Why evolution is not blind
“Learning theory is not just a different way of describing what Darwin already told us,” said Watson. “It expands what we think evolution is capable of. It shows that natural selection is sufficient to produce significant features of intelligent problem-solving.”
Conventionally, evolution, which depends on random variation, has been considered blind, or at least myopic, he notes. “But showing that evolving systems can learn from past experience means that evolution has the potential to anticipate what is needed to adapt to future environments in the same way that learning systems do.
“A system exhibits learning if its performance at some task improves with experience,” the authors note in the paper. “Reusing behaviors that have been successful in the past (reinforcement learning) is intuitively similar to the way selection increases the proportion of fit phenotypes [an organism’s observable characteristics or traits] in a population. In fact, evolutionary processes and simple learning processes are formally equivalent.
“In particular, learning can be implemented by incrementally adjusting a probability distribution over behaviors (e.g., Bayesian learning or Bayesian updating). Or, if a behavior is represented by a vector of features or components, by adjusting the probability of using each individual component in proportion to its average reward in past behaviors (e.g., Multiplicative Weights Update Algorithm, MWUA).”
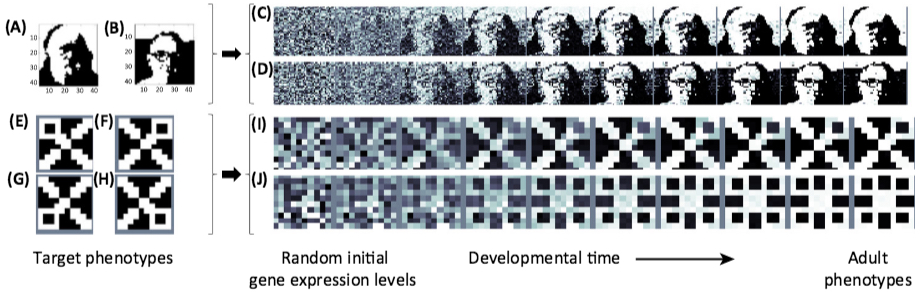
The evolution of connections in a Recurrent Gene Regulation Network (GRN) shows associative learning behaviors. When a Hopfield network is trained on a set of patterns with Hebbian learning, it forms an associative memory of the patterns in the training set. When subsequently stimulated with random excitation patterns, the activation dynamics of the trained network will spontaneously recall the patterns from the training set or generate new patterns that are generalizations of the training patterns. (A–D) A GRN is evolved to produce first one phenotype (set of characteristics or traits — Charles Darwin in this example) and then another (Donald Hebb) in an alternating manner. The resulting phenotype is not merely an average of the two phenotypic patterns that were selected in the past. Rather, different embryonic phenotypes (e.g., random initial conditions C and D) developed into different adult phenotypes (with this evolved GRN) and match either A or B. These two phenotypes can be produced from genotypes (DNA sequences) that are a single mutation apart. In a separate experiment, selection iterates over a set of target phenotypes (E–H). In addition to developing phenotypes that match patterns selected in the past (e.g., I), this GRN also generalizes to produce new phenotypes that were not selected for in the past but belong to a structurally similar class, for example, by creating novel combinations of evolved modules (e.g., developmental attractors exist for a phenotype with all four “loops” (J). This demonstrates a capability for evolution to exhibit phenotypic novelty in exactly the same sense that learning neural networks can generalize from past experience. (credit: Richard A. Watson and Eörs Szathmáry/Trends in Ecology and Evolution)
Unsupervised learning
An even more interesting process in evolution is unsupervised learning, where mechanisms do not depend on an external reward signal, the authors say in the paper:
By reinforcing correlations that are frequent, regardless of whether they are good, unsupervised correlation learning can produce system-level behaviors without system-level rewards. This can be implemented without centralized learning mechanisms. (Recent theoretical work shows that selection acting only to maximize individual growth rate, when applied to interspecific competition coefficients within an ecological community, produces unsupervised learning at the system level.)
This is an exciting possibility because it means that, despite not being a unit of selection, an ecological community might exhibit organizations that confer coordinated collective behaviors — for example, a distributed ecological memory that can recall multiple past ecological states. …
Taken together, correlation learning, unsupervised correlation learning, and deep correlation learning thus provide a formal way to understand how variation, selection, and inheritance, respectively, might be transformed over evolutionary time.
The authors’ new approach also offers an alternative to “intelligent design” (ID), which negates natural selection as an explanation for apparently intelligent features of nature. (The leading proponents of ID are associated with the Discovery Institute. See Are We Spiritual Machines? Ray Kurzweil vs. the Critics of Strong A.I.*** — a debate between Kurzweil and several Discovery Institute fellows.)
So if evolutionary theory can learn from the principles of cognitive science and deep learning, can cognitive science and deep learning learn from evolutionary theory?
* The authors are also affiliated with the Parmenides Foundation in Munich.
** Watson, R.A. et al. (2014) The evolution of phenotypic correlations and ‘developmental memory.’ Evolution 68, 1124–1138 and Pavlicev, M.et al. (2011) Evolution of adaptive phenotypic variation patterns by direct selection for evolvability. Proc. R. Soc. B Biol. Sci. 278, 1903–1912
*** This book is available free on KurzweilAI, as noted.
Abstract of How Can Evolution Learn?
The theory of evolution links random variation and selection to incremental adaptation. In a different intellectual domain, learning theory links incremental adaptation (e.g., from positive and/or negative reinforcement) to intelligent behaviour. Specifically, learning theory explains how incremental adaptation can acquire knowledge from past experience and use it to direct future behaviours toward favourable outcomes. Until recently such cognitive learning seemed irrelevant to the ‘uninformed’ process of evolution. In our opinion, however, new results formally linking evolutionary processes to the principles of learning might provide solutions to several evolutionary puzzles – the evolution of evolvability, the evolution of ecological organisation, and evolutionary transitions in individuality. If so, the ability for evolution to learn might explain how it produces such apparently intelligent designs.