Will this new ‘socially assistive robot’ from MIT Media Lab (or its progeny) replace teachers?
March 15, 2016
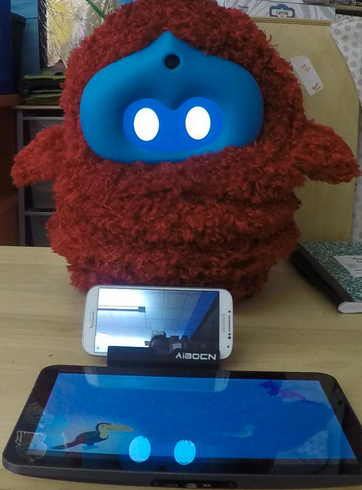
Tega, a socially assistive robot, is more fun than human teachers (credit: Personal Robots Group, MIT Media Lab)
Researchers in the Personal Robots Group at the MIT Media Lab, led by Cynthia Breazeal, PhD, have developed a powerful new “socially assistive” robot called Tega that senses the affective (emotional/feeling) state of a learner, and based on those cues, creates a personalized motivational strategy.
But what are the implications for the future of education … and society? (To be addressed in questions below.)
A furry, brightly colored robot, Tega is the latest in a line of smartphone-based, socially assistive robots developed in the MIT Media Lab. In a nutshell: Tega is fun, effective, and personalized — unlike many human teachers.
Breazeal and team say Tega was developed specifically to enable long-term educational interactions with children. It uses an Android device to process movement, perception and thinking and can respond appropriately to individual children’s behaviors — contrasting with (mostly boring) conventional education with its impersonal large class sizes, lack of individual attention, and proclivity to pouring children into a rigid one-size-fits-all mold.
The preschool classroom pilot
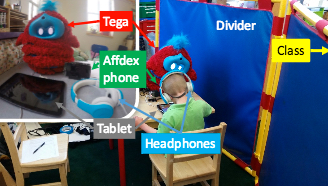
In the evaluation study, the child sat across the table from the robot. The tablet was placed on the table between them. The smartphone collecting data via Affdex sat beside the robot. The child wore headphones that were connected to the tablet and to the robot. (credit: Goren Gordon et al./AAAI)
The current version of Tega is now equipped with a second “AFFDEX” Android phone containing custom software developed by Affectiva Inc. — an NSF-supported spin-off of Rosalind Picard of MIT — that can interpret the emotional content of facial expressions, a method known as “affective computing” (see “Obama or Romney? Face-reading software monitors viewers’ responses to debate“).
Testing the setup in a preschool classroom, the researchers showed that the system can learn and improve itself in response to the unique characteristics of the students it worked with. It proved to be more effective at increasing students’ positive attitude towards the robot and activity than a non-personalized robot assistant.
The researchers piloted the system with 38 students aged three to five in a Boston-area school last year. Each student worked individually with Tega for 15 minutes per session over the course of eight weeks.
The students in the trial learned Spanish vocabulary from a tablet computer loaded with a custom-made learning game. Tega served not as a teacher but as a peer learner, encouraging students, providing hints when necessary and even sharing in students’ annoyance or boredom when appropriate. (Teaching vocabulary is an ineffective method for teaching languages, but lends itself to a controlled experiment.)
Personalizing responses
The system began by mirroring the emotional response of students — getting excited when they were excited, and distracted when the students lost focus — which educational theory suggests is a successful approach. However, it went further and tracked the impact of each of these cues on the student.
Over time, it learned how the cues influenced a student’s engagement, happiness, and learning successes. As the sessions continued, it ceased to simply mirror the child’s mood and began to personalize its responses in a way that would optimize each student’s experience and achievement.
Over the eight weeks, the personalization continued to increase. Compared with a control group that received only a mirroring reaction, students with the personalized response were (not surprisingly) more engaged by the activity, the researchers found.
“We know that learning from peers is an important way that children learn not only skills and knowledge, but also attitudes and approaches to learning such as curiosity and resilience to challenge,” says Breazeal, an associate professor of Media Arts and director of the Personal Robots Group at the MIT Media Laboratory.
“What is so fascinating is that children appear to interact with Tega as a peer-like companion in a way that opens up new opportunities to develop next-generation learning technologies that not only address the cognitive aspects of learning, like learning vocabulary, but the social and affective aspects of learning as well.”
The experiment served as a proof of concept for the idea of personalized educational assistive robots and also for the feasibility of using such robots in a real classroom. The system, which is almost entirely wireless and easy to set up and operate behind a divider in an active classroom, caused very little disruption and was thoroughly embraced by the student participants and by teachers.
“It was amazing to see,” said Goren Gordon, a visiting AI researcher who runs the Curiosity Lab at Tel Aviv University. “After a while, the students started hugging it, touching it, making the expression it was making and playing independently with almost no intervention or encouragement.”
The study showed the personalization process continued to progress even through the eight weeks, suggesting more time would be needed to arrive at an optimal interaction style. The researchers plan to improve upon and test the system in a variety of settings, including with students with learning disabilities, for whom one-on-one interaction and assistance is particularly critical and hard to come by.
“A child who is more curious is able to persevere through frustration, can learn with others and will be a more successful lifelong learner,” Breazeal says. “The development of next-generation learning technologies that can support the cognitive, social, and emotive aspects of learning in a highly personalized way is thrilling.”
The team reported its results at the 30th Association for the Advancement of Artificial Intelligence (AAAI) Conference in Phoenix, Arizona, in February. The work is supported by a five-year, $10 million Expeditions in Computing award from the National Science Foundation (NSF), which supports long-term, multi-institutional research in areas with the potential for disruptive impact.
Probing the uncertain future of socially assistive robots
Speaking of disruption, exactly where is this technology leading us? Don’t get me wrong — I totally love Tega and I want a Chinese version right now for studying Mandarin. But …
- Since socially assistive robots are apparently more fun (and presumably more effective at teaching) than humans, what are the possible effects on a child’s development, especially if implemented widely? Is it safe to exclude human teachers from learning?
- Could socially assistive robots lead to depersonalization — preferring robots and computers to human contact, especially contact with relatively aversive human teachers (who won’t have the patience of training to continually give students positive feedback like peer robots and will make demands on students, or punish them for not following instructions)? Will it cause future children to become functionally autistic?
- Could affective (emotion- and feelings-based) feedback from robots (or other devices) eventually lead to entraining a child’s (or adult’s) mind and eventually expose us to more powerful control by individualized, affective-based advertising, entertainment, media, and political and religious doctrine — and by charismatic psychopathic leaders*? It may be a small step from “assistive” to “controlling.”
- Will such hypothetical entrainment (or “entertrainment”) — enhanced by immersive (VR/AR) technologies and powerful social media — make us more likely to become (and even more) passive society that is highly influenced (or even controlled) by increasingly more intelligent machines that are also more fun and useful than people … or controlled (in proprietary versions) by those technologies’ makers, programmers, owners, or investors?
- What happens when future assistive robots, enhanced with deep learning and virtually omniscient access to information, replace the (current) 3.5 million full-time-equivalent elementary and secondary school teachers and the 1.3 million post-secondary teachers in the U.S. (and equivalents elsewhere in the world)?
- Such future enhanced assistive robots may have the ability to assume advanced animal, humanoid, alien, and other charismatic forms that could morph in real time and perform astounding, advanced theatrical events and even become an invisible part of our milieu — eventually becoming the dynamic real-time embodiment of an evolving, omnipresent/all-powerful intelligence that develops into superintelligence. Where does that take us?
* No reference to current political candidates implied. :)
MIT Media Lab Personal Robots Group | Tega: A Social Robot
MIT Media Lab Personal Robots Group | Learning a second language with a social assistive robot
Abstract of Affective Personalization of a Social Robot Tutor for Children’s Second Language Skills
Though substantial research has been dedicated towards using technology to improve education, no current methods are as effective as one-on-one tutoring. A critical, though relatively understudied, aspect of effective tutoring is modulating the student’s affective state throughout the tutoring session in order to maximize long-term learning gains. We developed an integrated experimental paradigm in which children play a secondlanguage learning game on a tablet, in collaboration with a fully autonomous social robotic learning companion. As part of the system, we measured children’s valence and engagement via an automatic facial expression analysis system. These signals were combined into a reward signal that fed into the robot’s affective reinforcement learning algorithm. Over several sessions, the robot played the game and personalized its motivational strategies (using verbal and non-verbal actions) to each student. We evaluated this system with 34 children in preschool classrooms for a duration of two months. We saw that children learned new words from the repeated tutoring sessions, the affective policy personalized to students over the duration of the study, and students who interacted with a robot that personalized its affective feedback strategy showed a signifi- cant increase in valence, as compared to students who interacted with a non-personalizing robot. This integrated system of tablet-based educational content, affective sensing, affective policy learning, and an autonomous social robot holds great promise for a more comprehensive approach to personalized tutoring.