Chips that mimic the brain in real time
July 24, 2013
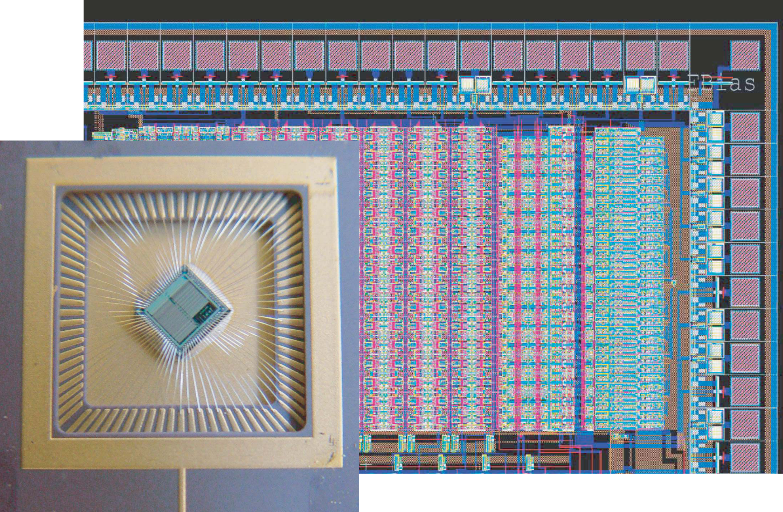
Photograph and layout of a multi-neuron chip comprising an array of analog/digital silicon neurons and synapse circuits, that can reproduce biophysically realistic neural response properties and dynamics in real-time. The chip was produced using a standard 0.35μm CMOS technology and it occupies an area of 10 square mm. It has 128 neuron circuits and 5120 synapse circuits. The neurons are connected to form a winner-take-all network, and the synapses implement realistic temporal dynamics as well as spike-timing dependent plasticity learning mechanisms. (Credit: University of Zurich)
Neuroinformatics researchers from the University of Zurich and ETH Zurich together with colleagues from the EU and U.S. have demonstrated how complex cognitive abilities can be incorporated into electronic systems made with “neuromorphic” chips.
They further show how to assemble and configure these electronic systems to function in a way similar to an actual brain.
No computer works as efficiently as the human brain — so building an artificial brain is the goal of many scientists. Neuroinformatics researchers from the University of Zurich and ETH Zurich say they have now made a breakthrough in this direction by understanding how to configure neuromorphic chips to imitate the brain’s information processing abilities in real time.
They demonstrated this by building an artificial sensory processing system that exhibits cognitive abilities.
Simulating biological neurons
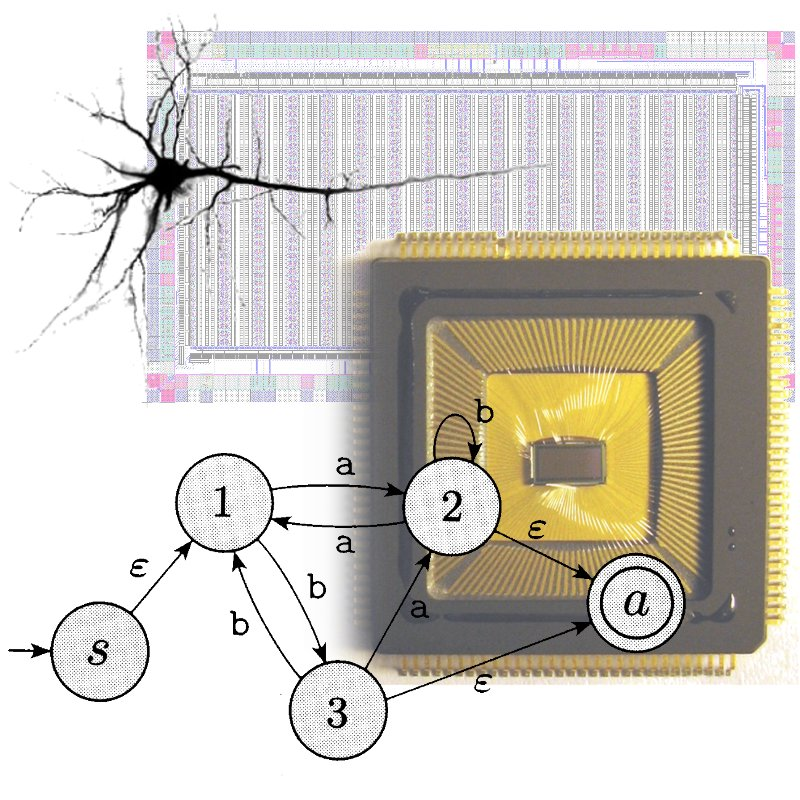
Image depicting a pyramidal neuron of cat visual cortex (top left), the layout of a neuromorphic multi-neuron chip (top right), the fabricated VLSI multi-neuron chip (bottom right), and an example of a finite-state-machine diagram that can be implemented by the method and hardware described in the PNAS article (credit: University of Zurich)
Most approaches in neuroinformatics are limited to the development of neural network models on conventional computers, or they aim to simulate complex nerve networks on custom-made VLSI systems or on supercomputers.
The Zurich researchers’ approach is to develop electronic circuits that are comparable to circuits in a real brain in terms of size, speed, and energy consumption.
“Our goal is to emulate the properties of biological neurons and synapses directly on microchips,” explains Giacomo Indiveri, a professor at the Institute of Neuroinformatics (INI), of the University of Zurich and ETH Zurich.
For example, an emulation of cortical circuits (see illustration to right).
The major challenge, says Indiveri, was to configure networks made of artificial (neuromorphic) neurons in such a way that they can perform specific tasks, which the researchers have now succeeded in doing:
So they developed a neuromorphic system that can carry out complex sensorimotor tasks in real time. They demonstrate a task that requires a short-term memory and context-dependent decision-making — typical traits that are necessary for cognitive tests.
In doing so, the INI team combined the neuromorphic neurons into networks that implemented neural processing modules equivalent to “finite-state machines” — a mathematical concept to describe logical processes or computer programs.
Behavior can be formulated as a “finite-state machine” and thus transferred to the neuromorphic hardware in an automated manner, says Indiveri. “The [machine] network connectivity patterns closely resemble structures that are also found in mammalian brains.
A real-time hardware neural-processing system
The scientists thus demonstrate how a real-time hardware neural-processing system, where the user dictates the behavior, can be constructed. “Thanks to our method, neuromorphic chips can be configured for a large class of behavior modes. Our results are pivotal for the development of new brain-inspired technologies,” Indiveri says.
One application, for instance, might be to combine the chips with sensory neuromorphic components, such as an artificial cochlea or retina, to create complex cognitive systems that interact with their surroundings in real time.

Real-time neuromorphic agent able to perform a context-dependent visual task. Two moving oriented bars are shown to an event-based 128×128 “silicon retina.” After processing, the “soft state machine” architecture is mapped onto the hardware neurons of the VLSI chips. (Credit: University of Zurich)
In the PNAS paper (see References below), the researchers demonstrate such a neuromorphic sensory agent: one that performs real-time context-dependent classification of motion patterns observed by a silicon retina.
Researchers at the Max Planck Institute for Brain Research and University of Bielefeld were also involved in this study.
Related workshops: The 2013 CapoCaccia Cognitive Neuromorphic Engineering Workshop and The Annual Telluride Workshop.
Comparisons with the Cornell–IBM SyNAPSE approach
In 2012, a Cornell–IBM SyNAPSE team fabricated a key building block of a modular neuromorphic architecture: a neurosynaptic core (steering a simulated robot around a virtual racetrack), as described in a news article in KurzweilAI. We asked Prof. Indiveri to comment on how these projects differ:
There are many common things between the IBM approach and ours. But there are also some fundamental differences. For example, IBM opted for a fully digital design approach, while we follow the original “neuromorphic engineering” approach proposed by Carver Mead in the early nineties and “listen to the silicon” (i.e., we use the physics of silicon to reproduce the biophysics of real neural circuits, and take the best of both analog and digital worlds). A more detailed story on this approach is here.
BTW, the silicon neurons and synapses in our chips share many similar features to those of the NeuroGrid chips (the Stanford large-scale neuromorphic system developed by Prof. Boahen). Both Boahen and I were at Caltech in the early to mid nineties, when Carver Mead was teaching at Caltech.
The main difference between our approach ant the IBM one (and the Stanford one as well for that matter), is that we use this technology as a medium for understanding the basic principles of (neural) computation.
We are not interested yet in building one-million-neuron artificial systems. Rather, we are trying to understand how to configure even relatively small networks of silicon neurons to achieve brain-like computation, including cognitive abilities, such as the ones demonstrated in our recent PNAS paper [see References below].
We study recurrent neural circuits and spike-based learning mechanisms that can lead to such models of computation, and implement them in analog/digital VLSI technology. — G. Indiveri